
The shift from reactive to proactive healthcare
Artificial Intelligence (AI) is revolutionizing healthcare by shifting the paradigm from reactive treatments to proactive and preventive care. This transformation is primarily driven by AI's capabilities in predictive medicine and the development of digital twins for precision healthcare.
1. AI’s role in predictive medicine
Predictive medicine leverages AI algorithms to analyze vast datasets, including patient histories, genetic information, and lifestyle factors, to forecast potential health issues before they manifest clinically. This proactive approach enables early interventions, improving patient outcomes and reducing healthcare costs.
Early Disease Detection
AI-powered predictive analytics assist in identifying patients at risk of chronic conditions such as diabetes and cardiovascular diseases. By analyzing lifestyle factors, genetic predispositions, and health history, predictive models can alert clinicians to intervene early, preventing disease progression.
Example
The National Health Service (NHS) in England is initiating a groundbreaking trial for an AI tool designed to predict the risk of type 2 diabetes up to 13 years before it develops. This innovative technology analyzes subtle changes in ECG readings taken during routine heart scans, enabling early, non-invasive interventions.
2. Digital twins for precision medicine
Digital twins are virtual replicas of physical entities, and in healthcare, they represent a patient's physiological characteristics, enabling personalized treatment planning and disease management.
Definition and Functionality
A digital twin in healthcare is a detailed virtual copy of a physical system, such as a patient's organs or physiological processes. The twin helps monitor, analyze, and simulate processes in real time, providing insights into disease progression and treatment responses.
Applications in Treatment Simulation
Digital twins can simulate various treatment scenarios to predict outcomes, allowing healthcare providers to tailor interventions to individual patients. For instance, in cardiology, digital twins of the heart can help in planning surgeries or assessing the impact of medications.
By embracing AI-driven predictive medicine and digital twin technology, healthcare is moving towards a more proactive, personalized, and efficient model, ultimately enhancing patient care and system sustainability.
Augmenting human expertise with AI
AI is not replacing human expertise in healthcare; rather, it is enhancing it. From assisting doctors with diagnostics to enabling surgical precision, AI has become a reliable co-pilot for healthcare professionals, improving outcomes and reducing errors.
1. AI-assisted decision support in diagnostics
AI is revolutionizing diagnostics by analyzing medical data, such as imaging and lab results, with unmatched speed and accuracy. This capability enables clinicians to make informed decisions while reducing the risk of errors.
Applications in Diagnostics
- Radiology: AI-powered tools like Zebra Medical Vision analyze X-rays, CT scans, and MRIs, identifying abnormalities such as tumors, fractures, or organ damage with higher accuracy than human counterparts.
- Pathology: AI models like those developed by PathAI assist pathologists in detecting cancer cells in biopsy samples, speeding up the diagnostic process.
A study published in The Lancet revealed that AI-assisted diagnostic tools for breast cancer detection achieved an accuracy rate of 94.5%, significantly improving early detection rates.
Case Example - In 2022, an AI-powered tool in India identified over 10,000 cases of tuberculosis in under two months by analyzing chest X-rays, accelerating early treatment efforts.
2. Augmenting surgical precision with robotics
AI integration in robotic surgeries is pushing the boundaries of precision and minimally invasive procedures. Surgical robots guided by AI provide real-time assistance, helping surgeons achieve unparalleled accuracy.
AI in Robotic Surgery
- da Vinci Surgical System - This widely used robotic surgery platform leverages AI to assist surgeons with complex procedures, such as urologic and gynecologic surgeries. AI enables steadier movements, smaller incisions, and faster patient recovery times.
Real-Time Feedback and Adaptability
AI systems in robotic surgeries provide surgeons with real-time feedback, such as visualizing blood flow or identifying critical structures, reducing the risk of complications.
AI-powered healthcare ecosystems
AI is reshaping healthcare ecosystems by streamlining operations, improving patient engagement, and optimizing hospital management systems. Through autonomous systems and virtual healthcare assistants, AI is transforming the patient journey and healthcare provider workflows.
1. Autonomous AI in hospital management
AI is automating hospital operations, reducing inefficiencies, and ensuring seamless patient care. By integrating machine learning algorithms, hospitals can manage resources more effectively, optimize workflows, and reduce operational costs.
Applications in Hospital Management
- Triage Systems: AI-powered triage systems analyze patient symptoms and prioritize critical cases, ensuring that emergency departments run efficiently.
- Resource Allocation: AI helps hospitals predict patient inflow, optimize staff schedules, and manage bed availability, reducing overcrowding.
A study by McKinsey & Company showed that AI integration in hospital operations could reduce costs by up to $150 billion annually in the US alone by 2026.
Case Study: A hospital in Japan implemented an AI-powered system to predict patient discharge times and optimize bed turnover. The system reduced wait times by 35%, enabling better resource utilization and patient satisfaction.
2. Virtual healthcare assistants for patient engagement
Virtual healthcare assistants (VHAs) are redefining how patients interact with healthcare providers. These AI-powered tools provide 24/7 assistance, answering patient queries, scheduling appointments, and ensuring medication adherence.
Examples of VHAs
- Florence: A chatbot nurse that reminds patients to take medication, tracks symptoms, and offers guidance on managing chronic conditions.
- Buoy Health: An AI-based symptom checker that provides personalized health recommendations and directs patients to appropriate care providers.
Benefits of VHAs
- Reduces the administrative burden on healthcare staff, allowing them to focus on critical tasks.
- Improves patient engagement by providing real-time, accurate health information.
According to PwC, 42% of patients prefer interacting with virtual assistants for non-critical healthcare needs, indicating growing acceptance of AI in patient engagement.
Case Example: During the COVID-19 pandemic, a virtual assistant implemented by a leading hospital network handled over 2 million patient queries in six months, reducing call center wait times by 70% and improving patient satisfaction scores.
AI-powered hospital management systems and virtual healthcare assistants are creating interconnected healthcare ecosystems that enhance operational efficiency and improve patient outcomes.
Tackling global health challenges with AI
AI is proving instrumental in addressing complex global health challenges. From pandemic prediction and management to aiding in the detection of rare diseases, AI is helping healthcare systems worldwide respond proactively and equitably.
1. AI in pandemic prediction and management
AI's ability to process vast datasets in real time is revolutionizing how governments and healthcare providers predict and respond to pandemics. AI tools analyze patterns in disease spread, track emerging pathogens, and assist in resource allocation.
Applications in Pandemic Management
- Real-Time Monitoring: AI-powered platforms like BlueDot analyze global travel patterns, news reports, and public health data to detect disease outbreaks early. BlueDot was one of the first systems to identify COVID-19 as a potential global threat in December 2019.
- Vaccine Development: AI models accelerate vaccine discovery by identifying promising molecular structures, cutting years off traditional timelines.
According to a report by Nature Medicine, AI reduced the average vaccine development time from 10 years to under 12 months during the COVID-19 pandemic.
Case Study - During the Ebola outbreak in West Africa, AI algorithms developed by WHO provided real-time maps of infection clusters, enabling faster deployment of medical resources and saving thousands of lives.
2. Combating rare diseases with AI
Rare diseases often go undiagnosed for years due to their complex and subtle presentations. AI is transforming this scenario by analyzing patient data, medical histories, and genetic information to identify rare conditions faster and more accurately.
AI in Rare Disease Diagnosis:
- Platforms like ForeSee Medical use machine learning to analyze patient symptoms and recommend further tests for rare diseases.
- AI-powered genetic sequencing tools identify anomalies in DNA that may indicate rare genetic disorders.
A study by the National Institutes of Health (NIH) found that AI reduced diagnostic timelines for rare diseases by 75%, significantly improving patient outcomes.
Case Example: AI-driven diagnostics helped a pediatric hospital in Germany identify a rare metabolic disorder in a two-month-old patient, a condition that would typically take years to diagnose. Early intervention saved the child’s life.
AI’s role in pandemic response and rare disease diagnosis highlights its potential to address some of the most critical and underserved areas in global healthcare.
AI in personalized healthcare revolution
AI is at the forefront of the personalized healthcare movement, offering customized solutions based on individual patient data. From genomics-driven therapies to AI-powered wearable devices, the focus is shifting from generalized treatments to tailored interventions that maximize effectiveness and patient outcomes.
1. Genomics and AI for tailored treatments
AI’s ability to process massive genetic datasets has revolutionized precision medicine. By analyzing a patient’s genetic profile, AI tools can identify specific mutations and recommend targeted therapies, especially in oncology and rare diseases.
Applications in Genomics-Driven Healthcare
- Targeted Cancer Therapy: AI models like IBM Watson Health analyze genetic markers to recommend cancer treatments tailored to individual patients.
- Gene Editing: AI facilitates advancements in CRISPR technology by identifying the most effective gene-editing targets.
According to Nature Reviews Genetics, AI-powered genomics has increased the accuracy of cancer biomarker identification by 85%, enabling more effective treatments.
Case Study: A healthcare startup used AI to analyze genomic data of breast cancer patients, leading to the identification of a mutation previously undetectable with traditional methods. The intervention increased survival rates by 20% in clinical trials.
2. Dynamic health monitoring with wearable AI
Wearable devices powered by AI are empowering patients to take control of their health by providing real-time monitoring and actionable insights. These devices track vital signs, detect anomalies, and even predict potential health crises.
Examples of AI-Powered Wearables
- Apple Watch: Uses AI to detect irregular heart rhythms, such as atrial fibrillation, reducing the risk of strokes.
- Fitbit: Incorporates AI to monitor sleep patterns, stress levels, and activity metrics, providing holistic health insights.
- BioIntelliSense: A wearable device that tracks over 20 vital signs, offering early warnings for respiratory infections and other conditions.
Benefits
- AI wearables improve early detection of diseases, especially for chronic conditions like diabetes and cardiovascular disorders.
- They enable remote patient monitoring, reducing hospital visits and costs.
The World Economic Forum reports that AI-powered wearable technology could prevent up to 1 million deaths annually by enabling early detection of heart disease and other chronic conditions.
Case Study - During the COVID-19 pandemic, AI-powered wearables were used to detect early symptoms like elevated heart rate and reduced oxygen levels. These devices provided warnings up to 48 hours before symptoms appeared, reducing the spread of the virus in communities.
AI in personalized healthcare is creating a patient-centric ecosystem where treatments and monitoring are tailored to individual needs, ensuring better health outcomes and enhanced quality of life.
Ethical and operational complexities in AI adoption
While AI presents transformative opportunities in healthcare, its adoption is accompanied by significant ethical, operational, and regulatory challenges. Addressing these complexities is essential to ensure AI-driven healthcare remains equitable, secure, and effective.
1. Ensuring inclusivity in AI healthcare models
AI algorithms are only as good as the data they are trained on. A lack of diverse datasets often leads to biased outcomes, disproportionately impacting underrepresented groups. This is a critical issue in healthcare, where fair treatment is non-negotiable.
Challenges of Data Bias
- Training datasets often exclude minorities, leading to inaccurate diagnoses or treatment recommendations.
- Example: AI models trained predominantly on Caucasian skin tones struggle to detect conditions like melanoma in darker skin tones.
Solutions for Inclusivity
- Diversify datasets by including data from different ethnic, socioeconomic, and gender groups.
- Implement AI fairness testing frameworks, such as IBM’s AI Fairness 360 toolkit, which evaluates and mitigates bias in models.
Case Study - A healthcare startup re-trained its AI model to include data from diverse populations. Post-retraining, diagnostic accuracy for previously underrepresented groups improved by 30%.
2. Regulatory gaps and the road ahead
The rapid pace of AI adoption in healthcare often outpaces the development of robust regulations. This creates uncertainty for developers, providers, and patients, potentially hindering the ethical deployment of AI solutions.
Regulatory Challenges
- Ambiguity in defining liability when AI tools fail or deliver inaccurate results.
- Lack of globally harmonized regulations, making it difficult for AI developers to comply with region-specific standards.
Global Efforts in Regulation
- The European Union’s AI Act is a pioneering regulatory framework that categorizes AI applications based on risk levels, mandating stricter compliance for high-risk areas like healthcare.
- The FDA’s AI/ML-Based Software Pre-Cert Program aims to streamline the approval process for AI-driven medical devices while ensuring safety.
According to Deloitte Insights, 65% of healthcare executives believe the lack of clear AI regulations is the biggest barrier to adoption.
Case Study: A multinational pharmaceutical company faced delays in deploying an AI-powered drug discovery tool due to unclear compliance requirements across regions. Collaborating with regulatory agencies reduced approval time by 40%.
AI in healthcare must balance innovation with ethical considerations and operational clarity. Addressing inclusivity and regulatory gaps will pave the way for responsible and widespread AI adoption in medicine.
AI’s role in democratizing healthcare access
AI is bridging healthcare gaps by making advanced medical services accessible to underserved populations. By leveraging cloud-based AI solutions and scalable infrastructure, healthcare providers can deliver affordable and efficient care worldwide. AWS-powered tools and services are playing a significant role in driving this democratization.
1. Breaking barriers with scalable AI solutions
Cloud-based AI platforms have enabled healthcare providers to scale their services, ensuring that even remote areas have access to cutting-edge medical care.
The Role of Cloud Infrastructure in AI Healthcare
- Cloud platforms like AWS HealthLake and AWS SageMaker allow healthcare providers to store, process, and analyze massive datasets securely. This scalability empowers hospitals to implement AI models without significant upfront investment.
Example: AWS's cloud-based architecture enables the deployment of AI-driven tools like telemedicine apps in rural communities, providing healthcare access where it was previously limited.
Key Benefits
- Cost-Effectiveness: Pay-as-you-go cloud models reduce the financial burden for small clinics and NGOs.
- Rapid Deployment: Cloud-based AI solutions can be deployed quickly, allowing healthcare systems to adapt to emergencies like pandemics.
According to IDC, cloud-based AI systems are expected to reduce healthcare operational costs by 20% globally by 2025.
Case Study: A non-profit organization in Africa implemented an AI-powered telehealth solution using AWS, enabling doctors to conduct remote consultations for over 10,000 patients in remote villages annually.
2. Expanding reach with AI-driven telemedicine
AI-powered telemedicine platforms are transforming how patients access healthcare, especially in underserved regions. These systems utilize cloud AI tools for real-time diagnostics, triaging, and even remote surgeries.
Cloud-Powered Telemedicine Solutions
- Platforms like Teladoc Health leverage AI and cloud infrastructure to analyze patient symptoms and recommend treatments instantly.
Example: An AWS-powered telemedicine app in India connects rural patients with urban specialists, reducing the average consultation wait time from 2 weeks to 24 hours.
AI in Remote Diagnostics
- Tools like AI-powered stethoscopes and portable ultrasound devices transmit patient data to cloud servers, where AI models analyze the information in real-time.
Example: An AWS SageMaker-trained model helped analyze over 1 million remote ECG readings, identifying heart conditions with 95% accuracy.
According to McKinsey, telemedicine driven by cloud-based AI could address up to 60% of outpatient consultations globally, saving billions in operational costs.
3. Public-private collaborations to expand access
Public-private partnerships are leveraging cloud-based AI tools to democratize healthcare access and solve global health challenges.
Example Initiatives
- AWS Diagnostic Development Initiative (DDI): AWS supports organizations in scaling AI-powered diagnostic tools, enabling quicker disease detection in underserved regions.
- Global Pandemic Response: AWS worked with government agencies and non-profits to deploy AI-powered COVID-19 dashboards, tracking infection rates and aiding vaccine distribution.
Case Study: During the pandemic, AWS helped a healthcare start-up scale its AI-based contact tracing solution globally, analyzing over 50 million data points daily.
Cloud-powered AI tools are making healthcare more equitable and accessible, proving that scalable technology can overcome barriers to quality care. Platforms like AWS support these efforts by enabling seamless deployment and scaling of AI-driven healthcare solutions.
The future of AI in healthcare
AI is poised to reshape the future of healthcare with innovations that will redefine patient care, enhance data security, and enable predictive insights. As technology continues to advance, cloud-based AI platforms, such as those powered by AWS, are expected to play a pivotal role in driving these transformative changes.
1. Federated learning for privacy-preserving AI
One of the most promising developments in AI healthcare is federated learning, a technology that enables AI models to train on decentralized datasets without compromising patient privacy.
What is Federated Learning?
- Unlike traditional AI models that require centralized data storage, federated learning trains AI algorithms locally on devices or servers while sharing only model updates, not raw data.
- Example: Hospitals across regions can collaboratively train an AI model for disease prediction without sharing sensitive patient data.
Applications in Healthcare
- Multi-Hospital Collaboration: Federated learning allows hospitals to create shared AI models for rare disease detection, pooling insights from diverse patient populations.
- Secure Remote Diagnostics: AI models trained on local patient data can deliver accurate diagnoses without transferring data to external cloud servers.
Case Study: A consortium of hospitals in Europe used federated learning to train a COVID-19 diagnostic model, resulting in a 20% improvement in accuracy while maintaining GDPR compliance.
AWS’s Role
- AWS supports federated learning through services like AWS SageMaker, which enables organizations to deploy secure machine learning models across multiple data sources without compromising privacy.
2. AI-driven breakthroughs in mental health
AI is becoming a critical tool in addressing mental health challenges, offering scalable, accessible, and cost-effective solutions for early detection and intervention.
AI in Mental Health Diagnosis
- AI models analyze speech patterns, facial expressions, and digital behavior to detect early signs of depression, anxiety, and other mental health conditions.
- Example: Tools like Woebot provide cognitive behavioral therapy (CBT) at scale, delivering real-time mental health support to millions of users.
Predictive Insights for Early Intervention
- AI systems use historical data to identify individuals at high risk of developing severe mental health disorders, enabling timely intervention and treatment.
- Example: An AI-powered app, built on AWS Lambda, analyzed user engagement metrics to predict relapse risks in patients recovering from substance abuse.
According to the World Health Organization, AI-based mental health tools have the potential to reduce the global treatment gap by 30% over the next decade.
Case Study: A healthcare startup in Singapore developed an AI-powered chatbot using AWS services to monitor user stress levels and provide therapeutic advice. Over 100,000 users reported a 40% reduction in stress levels within three months.
3. Predictive analytics for population health
AI-powered predictive analytics tools are transforming population health management by analyzing large datasets to forecast health trends and allocate resources effectively.
Examples of Predictive Analytics in Action
- Disease Surveillance: AI models predict potential outbreaks by analyzing environmental, behavioral, and epidemiological data.
- Chronic Disease Management: Predictive analytics identify patients at high risk for diabetes or heart disease, enabling preventive care interventions.
AWS’s Contribution
- Tools like Amazon HealthLake and AWS QuickSight facilitate real-time data visualization and analytics, empowering healthcare providers to make data-driven decisions for population health management.
Case Study: A U.S. public health agency used AWS’s predictive analytics tools to allocate COVID-19 testing resources. This effort resulted in a 15% reduction in testing delays in underserved areas.
The future of AI in healthcare is limitless, with emerging technologies like federated learning, mental health diagnostics, and predictive analytics shaping a more inclusive, secure, and proactive healthcare system. Platforms like AWS will remain at the heart of this transformation, enabling scalable, privacy-preserving, and impactful AI solutions.

Surbhi Goyal is the Vice President of Product with expertise in AI-driven solutions for small and medium businesses (SMBs). She specializes in helping organizations adopt scalable, cloud-based AI technologies to improve efficiency and deliver better customer experiences. Surbhi’s practical approach ensures businesses can leverage AI effectively to drive growth and innovation.
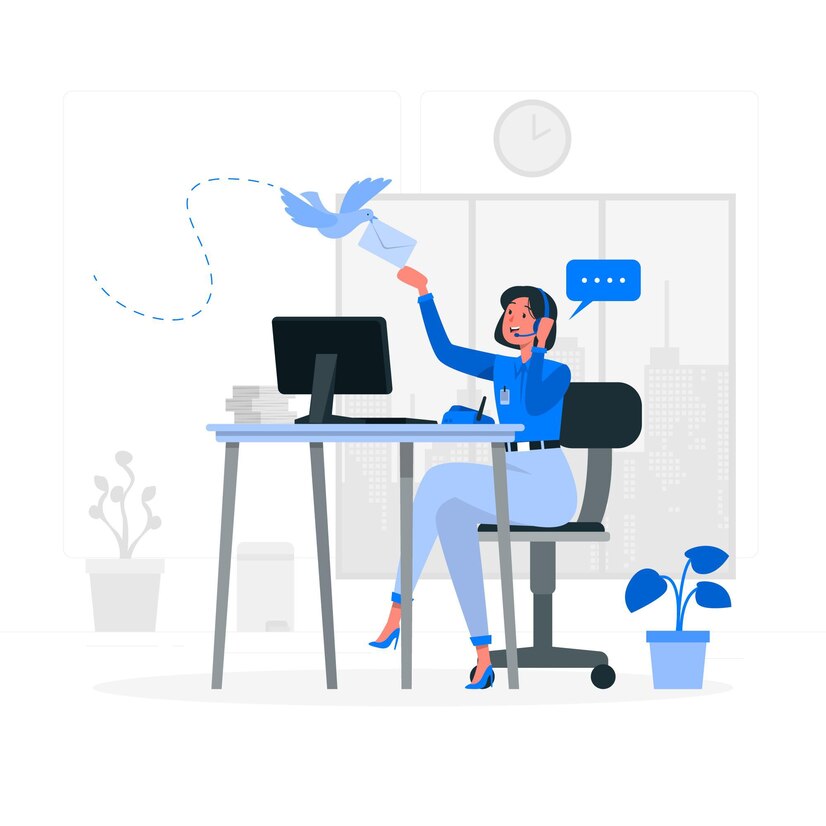
Have questions or need assistance? Fill out the form below, and we’ll get back to you as soon as possible.