
1. The rise of AI in modern supply chains
The global supply chain landscape is undergoing a rapid transformation, fueled by the power of artificial intelligence (AI). Traditionally, supply chains have relied on manual processes, static forecasts, and reactive decision-making. However, with increasing market volatility, labor shortages, and rising customer expectations, businesses can no longer afford inefficiencies. AI is emerging as the key enabler of intelligent, automated, and predictive supply chains that enhance resilience, reduce costs, and improve operational efficiency.
How AI is revolutionizing supply chain management
AI’s integration into supply chain operations is reshaping logistics, procurement, inventory management, and demand forecasting. By leveraging machine learning algorithms, predictive analytics, and automation, businesses can shift from reactive supply chain management to proactive decision-making. This results in:
- Faster response times to supply chain disruptions
- Optimized inventory levels with demand forecasting
- Reduced operational costs through automation
- Improved sustainability with AI-driven resource optimization
Historical perspective: from traditional logistics to intelligent automation
Historically, supply chains relied on manual record-keeping, paper-based logistics, and static planning models that lacked flexibility. The shift to Enterprise Resource Planning (ERP) systems in the 1990s allowed businesses to digitize supply chain data, but challenges remained. With the rise of big data, cloud computing, and AI-driven analytics, supply chains are now transitioning towards intelligent automation, where AI continuously learns and adapts to market conditions.
Milestones in supply chain transformation:
- 1990s – Digitization of logistics with ERP systems
- 2000s – Integration of e-commerce and globalized supply chains
- 2010s – Cloud computing and IoT-enabled tracking systems
- 2020s – AI-driven predictive analytics, automation, and self-optimizing supply chains
The growing reliance on ai for predictive analytics, automation, and decision-making
A Gartner report forecasts that by 2026, 75% of large enterprises will have adopted AI-powered supply chain analytics, enabling them to reduce forecasting errors by 50% (Gartner). AI allows businesses to:
- Predict demand fluctuations and adjust procurement strategies accordingly
- Automate routine supply chain tasks, reducing human intervention and errors
- Analyze vast amounts of supply chain data to improve efficiency and identify risks
- Enhance logistics and transportation efficiency through route optimization algorithms
Why AI is critical for global supply chain resilience
The COVID-19 pandemic, geopolitical tensions, and natural disasters have exposed the fragility of traditional supply chains. AI is playing a crucial role in building supply chain resilience by:
- Improving real-time visibility across suppliers, manufacturers, and distributors
- Detecting early warning signals of potential supply disruptions
- Optimizing procurement strategies to diversify suppliers and reduce dependency risks
- Enhancing agility in responding to sudden market changes
According to McKinsey, AI-driven supply chain optimization can reduce operational costs by up to 20% and improve service levels by 10-15% (McKinsey).
2. The biggest supply chain challenges and how AI is solving them
The supply chain industry is facing unprecedented challenges due to global disruptions, increasing customer expectations, and operational inefficiencies. AI is emerging as a critical solution to optimize processes, enhance agility, and improve decision-making in supply chain management. This section explores the major pain points in traditional supply chains and how AI-powered solutions are transforming them.
2.1 Supply chain disruptions: volatility, uncertainty, and global risks
Global supply chains are highly interconnected and complex, making them vulnerable to:
- Geopolitical tensions (e.g., trade wars, import/export restrictions)
- Natural disasters disrupting logistics and production
- Pandemics affecting supply chain workforce availability
- Economic fluctuations impacting demand and supply stability
How AI mitigates disruptions
AI-powered predictive analytics and risk assessment models can proactively identify potential disruptions before they occur. By analyzing historical data, weather patterns, market trends, and geopolitical conditions, AI can:
- Provide early warnings for supply chain risks
- Suggest alternative suppliers and logistics routes
- Optimize inventory levels to buffer against demand fluctuations
According to Gartner, by 2026, 60% of supply chain organizations will invest in AI-driven risk management to improve resilience (Gartner).
2.2 Inefficient demand forecasting: overstocking vs. stockouts
One of the biggest issues in supply chain management is inaccurate demand forecasting, leading to:
- Excess inventory that increases holding costs
- Stockouts, causing revenue losses and dissatisfied customers
- Bullwhip effect, where small demand fluctuations cause massive supply chain imbalances
How AI improves demand forecasting
AI-driven machine learning algorithms analyze historical sales data, customer trends, seasonality, and real-time market insights to generate highly accurate demand predictions. Key benefits include:
- Optimized inventory levels to reduce waste and costs
- Dynamic pricing strategies based on real-time demand fluctuations
- Automated supply chain adjustments to prevent overstocking or shortages
McKinsey reports that AI-enhanced demand forecasting can reduce forecasting errors by up to 50%, leading to 20-30% reductions in inventory costs (McKinsey).
2.3 Supply chain visibility: lack of end-to-end tracking
Many businesses struggle with limited visibility into their supply chain operations, making it difficult to track:
- Supplier reliability and performance
- Real-time shipment locations
- Production bottlenecks
- Inventory movement across warehouses
How AI enhances supply chain visibility
AI-powered real-time tracking and IoT integration allow businesses to:
- Monitor shipments and inventory in real-time
- Predict potential delays or disruptions before they impact operations
- Automate supplier performance analytics to enhance collaboration
A PwC survey found that 61% of supply chain executives consider AI-driven visibility solutions essential for optimizing operations (PwC).
2.4 Logistics and transportation inefficiencies
Traditional logistics and transportation processes suffer from:
- High fuel and operational costs
- Inefficient delivery routes
- Lack of real-time fleet monitoring
- Delays due to unpredictable factors like traffic and weather
How AI optimizes logistics and transportation
AI-powered route optimization algorithms and fleet management solutions enhance logistics efficiency by:
- Reducing fuel consumption and operational costs
- Identifying optimal delivery routes based on real-time traffic and weather conditions
- Automating warehouse operations to improve order fulfillment speed
According to DHL, AI-powered logistics can reduce delivery times by 20% and cut fuel costs by 15% (DHL Logistics).
2.5 Cybersecurity risks in supply chain data management
As supply chains become increasingly digitized, they are more vulnerable to cyberattacks. Risks include:
- Data breaches exposing supplier and customer information
- Ransomware attacks disrupting operations
- Lack of secure data sharing between supply chain partners
How AI strengthens supply chain cybersecurity
AI-driven cybersecurity solutions can:
- Detect anomalies in network activity to identify potential cyber threats
- Automate threat response mechanisms to mitigate security breaches in real-time
- Enhance encryption and authentication protocols for secure data sharing
According to IBM, AI-powered cybersecurity can reduce supply chain security breaches by 40% through automated risk detection (IBM Security).
With AI addressing these critical pain points, the next section will explore real-world use cases of AI in supply chain management and how leading enterprises are leveraging AI for efficiency and innovation.
3. Real-world use cases of AI in supply chain management
Artificial intelligence is already revolutionizing supply chain operations across industries, helping businesses optimize logistics, reduce costs, and improve decision-making. This section explores real-world AI applications in supply chain management, illustrating how leading enterprises leverage AI to drive efficiency and innovation.
3.1 AI-powered demand forecasting: anticipating market needs with precision
Many companies struggle with inaccurate demand predictions, leading to inventory shortages or excess stock. AI-driven demand forecasting uses machine learning algorithms to analyze historical data, customer behavior, and external market factors to predict future demand more accurately.
Case study: Walmart’s -driven inventory optimization
- Walmart leverages AI-powered predictive analytics to track purchasing trends and forecast demand fluctuations across its global supply chain.
- Using real-time data from point-of-sale systems, weather forecasts, and economic trends, Walmart’s AI models adjust inventory distribution dynamically.
- As a result, Walmart has achieved 30% lower inventory costs and 90% improvement in stock replenishment accuracy (Forbes).
How businesses can implement this
Companies can integrate AI-powered demand forecasting through solutions like AWS Forecast, which uses machine learning models to optimize supply chain planning. Businesses can also leverage AI and data services to develop custom predictive analytics solutions tailored to their needs.
3.2 Warehouse automation with AI-driven robotics
Traditional warehouses rely on manual labor, which increases operational costs and processing times. AI-powered robotics and computer vision are transforming warehouse operations by automating sorting, picking, and inventory tracking.
Amazon’s AI-powered fulfillment centers
- Amazon’s fulfillment centers use AI-powered robots and computer vision systems to automate order processing.
- Kiva robots transport inventory shelves, reducing the time warehouse workers spend searching for items.
- AI-driven computer vision systems track product movements and optimize storage layouts for maximum efficiency.
- This has led to a 40% reduction in warehouse operational costs and 50% faster order fulfillment times (CNBC).
3.3 AI-driven logistics and route optimization
Inefficient logistics and transportation routes lead to higher fuel costs, delays, and poor delivery performance. AI-powered route optimization software uses real-time traffic, weather, and delivery constraints to determine the most efficient transportation routes.
DHL’s AI-powered smart routing system
- DHL uses AI-powered smart routing to optimize package deliveries across its global logistics network.
- By integrating real-time GPS data, weather patterns, and delivery schedules, AI automatically adjusts routes to minimize delays.
- This approach has resulted in 20% lower transportation costs and 15% faster delivery times (DHL Logistics).
3.4 AI-powered supplier risk management
Many businesses lack visibility into supplier performance, which increases risks such as delayed shipments, compliance issues, and product defects. AI-driven risk management tools analyze supplier history, financial stability, and compliance records to predict potential risks before they affect supply chains.
Siemens AI-driven supplier risk analysis
- Siemens leverages AI-powered supplier risk management to assess supplier performance based on historical transaction data, market reports, and geopolitical risks.
- AI models assign risk scores to suppliers and automatically suggest alternative suppliers if risks exceed predefined thresholds.
- This system has helped Siemens reduce supplier-related disruptions by 35% and improve procurement efficiency (Siemens).
3.5 AI-powered sustainability and carbon footprint tracking
Sustainability is a growing concern in supply chain management. Many companies are now using AI-powered sustainability tracking to measure carbon emissions, energy consumption, and waste management.
Unilever’s AI-driven sustainability monitoring
- Unilever utilizes AI-powered analytics to track carbon footprint metrics across its global supply chain.
- AI models analyze energy consumption, material sourcing, and transportation emissions to suggest eco-friendly alternatives.
- The initiative has helped Unilever cut carbon emissions by 25% and improve sustainability compliance (World Economic Forum).
4. Implementing AI in supply chain management: strategies for success
While AI is transforming supply chain management, successful implementation requires clear strategies, robust infrastructure, and industry best practices. Businesses must align AI initiatives with supply chain goals, ensure seamless integration with existing systems, and address common adoption challenges.
This section outlines key strategies for implementing AI in supply chains, ensuring businesses can maximize AI’s benefits while mitigating risks.
4.1 Aligning AI initiatives with business objectives
AI adoption should align with an organization’s supply chain goals, whether it’s reducing costs, improving efficiency, enhancing sustainability, or optimizing risk management. Companies must define clear KPIs and measurable outcomes before integrating AI into their supply chain operations.
Best practices
- Identify specific pain points AI can solve, such as demand forecasting, logistics optimization, or supplier risk analysis.
- Set quantifiable success metrics, such as 20% reduction in inventory waste, 30% faster delivery times, or 40% improvement in supplier performance.
- Prioritize AI use cases that provide quick wins, such as automating repetitive manual tasks or using predictive analytics for inventory management.
For example: Tesla’s AI-driven supply chain optimization
- Tesla integrates AI-powered demand forecasting with real-time production analytics to optimize material procurement and reduce supply chain disruptions.
- AI-driven predictive maintenance on Tesla’s Gigafactories ensures uninterrupted production, leading to 25% reduction in unplanned downtime (McKinsey).
4.2 Integrating AI with existing supply chain systems
A major challenge for businesses is seamlessly integrating AI into their existing supply chain management (SCM) platforms. AI models must work alongside ERP, CRM, and logistics management systems to drive actionable insights and automated decision-making.
Key integration strategies
- Cloud-based AI solutions: Hosting AI models on AWS, Google Cloud, or Microsoft Azure ensures scalability and easy integration with SCM platforms like SAP, Oracle SCM, and Microsoft Dynamics.
- API-driven connectivity: Using AI-powered APIs to connect predictive analytics tools with inventory and warehouse management systems.
- IoT integration: Combining AI with IoT sensors to collect real-time logistics, warehouse, and transportation data for improved decision-making.
Maersk’s AI-driven logistics automation
- Maersk, one of the world’s largest shipping companies, integrates AI-powered analytics with IoT to monitor real-time cargo movement, predict delays, and optimize global shipping routes.
- This has led to a 15% reduction in fuel consumption and 30% improvement in on-time deliveries (IBM).
4.3 Overcoming adoption challenges in AI-powered supply chains
Despite AI’s potential, many businesses face barriers to adoption, such as high implementation costs, data silos, lack of technical expertise, and regulatory concerns. Addressing these challenges is crucial for AI’s long-term success in supply chain operations.
Common challenges & solutions
High implementation costs
- Solution: Use AI-as-a-Service (AIaaS) platforms like AWS AI Services to access scalable, pay-as-you-go AI solutions.
Data quality & integration issues
- Solution: Implement data lakes and AI-driven data pipelines to clean, unify, and structure fragmented supply chain data.
Lack of AI expertise
- Solution: Partner with AI consulting firms or cloud providers to develop AI solutions without requiring in-house AI teams.
Regulatory compliance & security concerns
- Solution: Use AWS AI governance tools and compliance frameworks to ensure adherence to global supply chain regulations.
Nestlé’s AI-powered supply chain risk management
- Nestlé integrates AI-powered risk analysis tools to predict supply chain disruptions caused by geopolitical tensions, climate risks, and supplier financial instability.
- AI models analyze third-party risk databases and historical supplier performance to proactively adjust procurement strategies, reducing risk exposure by 40% (Deloitte).
4.4 Scaling AI-driven supply chains for future growth
To stay competitive, businesses must continuously evolve AI-driven supply chain solutions by integrating advanced AI capabilities, such as generative AI, deep learning, and autonomous decision-making systems.
Future-proofing AI-driven supply chains
- Generative AI for automated procurement: AI models will generate real-time procurement recommendations, reducing reliance on manual supplier negotiations.
- Autonomous supply chain decision-making: AI-powered digital twins will simulate supply chain operations to identify bottlenecks, inefficiencies, and risks.
- AI-powered sustainability tracking: Businesses will integrate AI-driven carbon footprint tracking systems to achieve sustainable supply chain operations.
5. Future trends in AI-powered supply chain management
The next decade will witness AI revolutionizing supply chain management at an unprecedented scale. With generative AI, autonomous supply chains, and sustainability-driven logistics at the forefront, businesses must prepare for a fully AI-integrated supply chain ecosystem.
This section explores emerging trends that will shape the future of AI in supply chain management, providing insights into how businesses can stay ahead in an AI-driven world.
5.1 Generative AI: revolutionizing predictive supply chain analytics
Generative AI is set to redefine supply chain forecasting, planning, and risk mitigation. Unlike traditional AI models, which analyze past data, generative AI can create new supply chain models, simulate future demand scenarios, and optimize procurement strategies.
How generative AI is transforming supply chain analytics
- Autonomous demand forecasting: AI models generate real-time demand forecasts, allowing businesses to adjust procurement and inventory in response to market shifts.
- AI-powered supplier negotiations: Generative AI will create dynamic procurement strategies by analyzing supplier market trends, cost structures, and risk factors.
- Automated scenario planning: AI will simulate what-if supply chain scenarios, identifying potential bottlenecks and alternative solutions before disruptions occur.
5.2 Autonomous supply chains: the rise of self-learning logistics
AI is paving the way for fully autonomous supply chains, where self-learning systems handle logistics, warehouse management, and order fulfillment with minimal human intervention.
Key innovations driving autonomous supply chains
- AI-powered warehouse robotics: Automated AI-driven robots will manage inventory, pack shipments, and streamline fulfillment.
- Self-optimizing logistics networks: AI will continuously learn and optimize delivery routes, reducing transportation costs and cutting carbon emissions.
- AI-driven fleet management: AI will predict vehicle maintenance needs, preventing breakdowns and ensuring on-time deliveries.
Amazon’s autonomous warehouse logistics
- Amazon uses AI-powered robotics to automate warehouse inventory management, cutting order processing times by 50% and reducing operational costs by 30% (McKinsey).
5.3 AI-powered sustainability and green supply chains
As businesses strive to meet sustainability goals, AI is emerging as a critical enabler for eco-friendly supply chains. AI-powered sustainability tools will optimize energy consumption, reduce waste, and enable carbon-neutral logistics.
How AI is driving supply chain sustainability
- AI-driven carbon footprint tracking: Businesses can use AI-powered analytics to monitor and reduce emissions across supply chain operations.
- Sustainable inventory optimization: AI will minimize overproduction and excess inventory, reducing waste and improving resource efficiency.
- AI-powered reverse logistics: AI will enhance product returns and recycling processes, ensuring supply chains align with circular economy principles.
Unilever’s AI-driven green supply chain
- Unilever integrates AI with real-time sustainability data to reduce its supply chain emissions by 25%, optimizing sourcing strategies and reducing waste production (World Economic Forum).
5.4 Blockchain and AI: the future of transparent supply chains
The integration of AI with blockchain technology will enhance supply chain transparency, security, and traceability, reducing fraud and ensuring compliance.
Key benefits of AI-powered blockchain supply chains
- Real-time supply chain visibility: AI-driven blockchain records every transaction, ensuring end-to-end transparency.
- Fraud detection and compliance: AI identifies anomalies in supply chain transactions, preventing counterfeiting and fraud.
- AI-driven contract automation: Smart contracts powered by AI will automate supplier agreements and logistics tracking.
IBM’s AI-powered blockchain for food supply chains
- IBM’s Food Trust blockchain, powered by AI, ensures real-time traceability of food products, reducing contamination risks and cutting food waste by 40% (IBM).
6. Future-proofing supply chains with AI
The evolution of AI-powered supply chain management is no longer a vision for the future—it is a present-day imperative. Businesses that embrace AI-driven automation, predictive analytics, and sustainable logistics will gain a significant competitive edge, while those that hesitate risk falling behind in an increasingly digitized global marketplace.
This final section summarizes the key takeaways and provides actionable steps for organizations looking to leverage AI in their supply chains.
6.1 Why AI is the future of supply chain management
- AI is enabling predictive, self-optimizing supply chains: Companies integrating AI-powered analytics are achieving 30% higher operational efficiency (McKinsey).
- Generative AI is redefining supply chain forecasting: Businesses leveraging AI-powered demand prediction models reduce overstock by 40% and enhance inventory accuracy.
- Autonomous supply chains are emerging as the new standard: AI-driven automation in warehousing and logistics is expected to cut supply chain costs by 50% in the next five years (Forbes).
- Sustainability in supply chains is no longer optional: AI-powered tracking and optimization tools are helping companies reduce emissions and meet global sustainability mandates (World Economic Forum).
- Blockchain and AI are securing supply chain transactions: By integrating AI with blockchain, businesses are improving fraud detection, compliance, and real-time tracking of goods (IBM).
AI-driven supply chain management is no longer about keeping up with the competition—it is about leading the industry with smarter, more efficient, and future-ready logistics.
6.2 How businesses can take action today
Step 1: Assess AI Readiness
- Conduct an internal audit to identify manual processes that can be automated with AI.
- Evaluate existing data infrastructure to ensure seamless AI adoption.
- Identify supply chain inefficiencies that AI-powered solutions can resolve.
Step 2: Implement AI-Driven Analytics & Automation
- Integrate predictive analytics models for demand forecasting and inventory management.
- Deploy AI-powered robotics and warehouse automation to optimize logistics operations.
- Leverage generative AI for scenario planning and supply chain risk mitigation.
Step 3: Future-Proof Supply Chains with AI & Cloud Services
- Adopt AI-powered sustainability tracking tools to align with ESG goals.
- Secure supply chain transactions using AI-driven blockchain transparency.
- Move supply chain operations to cloud-based infrastructure for scalability and resilience.
6.3 AI-driven supply chain excellence
AI in supply chain management is no longer an experimental technology—it is an operational necessity. Companies that embrace AI-powered automation, predictive intelligence, and sustainability tracking will gain cost efficiencies, greater resilience, and a competitive market advantage.
With AI evolving at an accelerated pace, the future supply chain will be:
- Self-learning and autonomous
- Predictive and responsive to market fluctuations
- Digitally connected across ecosystems
- Sustainable and AI-optimized for efficiency
The time to act is now. Businesses that start their AI-powered supply chain transformation today will be the industry leaders of tomorrow.

Surbhi Goyal is the Vice President of Product with expertise in AI-driven solutions for small and medium businesses (SMBs). She specializes in helping organizations adopt scalable, cloud-based AI technologies to improve efficiency and deliver better customer experiences. Surbhi’s practical approach ensures businesses can leverage AI effectively to drive growth and innovation.
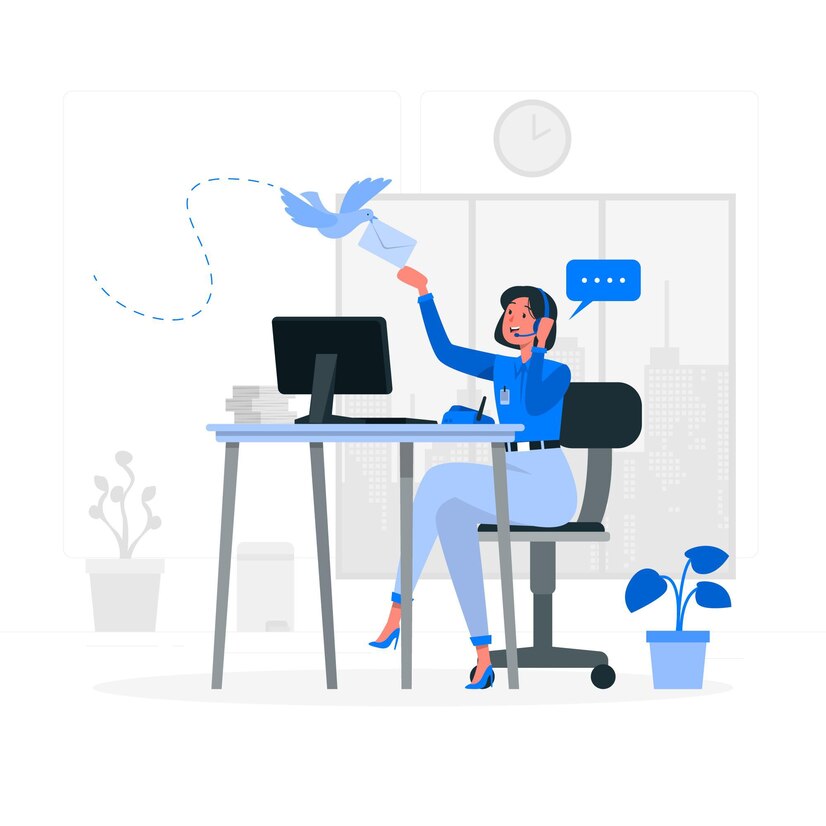
Have questions or need assistance? Fill out the form below, and we’ll get back to you as soon as possible.