
1. The new era of intelligent maintenance
Overview of predictive maintenance and its growing role in modern industries
Predictive maintenance is revolutionizing how industries manage assets, reducing unexpected failures and optimizing operational efficiency. Unlike traditional maintenance approaches, predictive maintenance leverages data analytics, IoT sensors, and AI-driven models to anticipate potential failures before they happen. This shift is helping industries minimize downtime, cut maintenance costs, and enhance asset longevity.
According to a McKinsey report, industries implementing predictive maintenance have reported:
- 20–30% cost savings on maintenance operations
- Up to 50% reduction in machine downtime
- 25% extension in equipment lifespan
How data analytics is transforming traditional maintenance models
Historically, companies relied on reactive or preventive maintenance models:
- Reactive Maintenance: Fixing machines after they fail, leading to costly downtimes.
- Preventive Maintenance: Performing maintenance at scheduled intervals, sometimes resulting in unnecessary servicing.
With predictive maintenance, AI-powered analytics assess real-time data from sensors to predict exactly when maintenance is needed, preventing failures while reducing maintenance costs. Companies can now analyze massive datasets from industrial equipment to forecast breakdowns weeks or even months in advance.
For example, General Electric’s (GE) Predix platform uses predictive maintenance analytics to monitor wind turbines and jet engines in real-time, helping reduce costly failures by up to 50%.
Global market trends and adoption statistics of predictive maintenance
The global predictive maintenance market is growing rapidly as industries increasingly rely on AI-driven solutions:
- The predictive maintenance market is expected to reach $23.5 billion by 2025, growing at a CAGR of 25.2%.
- By 2026, over 80% of industrial companies will integrate predictive analytics into their maintenance strategies.
- Airlines using predictive maintenance have reduced unscheduled maintenance events by 30%, significantly improving safety and reliability.
Key benefits: reduced downtime, cost savings, and asset longevity
Industries adopting predictive maintenance see multiple business advantages, including:
- Lower operational costs by reducing unnecessary maintenance.
- Maximized uptime with fewer machine failures and improved efficiency.
- Longer asset lifespan by detecting potential faults early.
- Enhanced safety and compliance through proactive maintenance scheduling.
For example, Siemens uses AI-powered predictive maintenance in its manufacturing plants to reduce breakdowns by 40%, saving millions in repair costs.
2. The science behind predictive maintenance: how data analytics drives precision
Understanding the predictive maintenance framework
Predictive maintenance operates at the intersection of IoT, AI, cloud computing, and big data analytics. It uses advanced machine learning models to process vast amounts of real-time sensor data, helping businesses detect early signs of equipment failure before they disrupt operations.
A typical predictive maintenance framework includes:
- Data Collection: IoT sensors and monitoring devices gather real-time data on machine performance (temperature, vibration, pressure, etc.).
- Data Storage & Processing: Cloud platforms, such as AWS IoT and Azure, store and analyze data.
- Predictive Analytics & AI Models: Machine learning algorithms identify patterns and anomalies.
- Actionable Insights: Businesses receive alerts on potential failures, allowing them to take preventive actions.
Role of IoT, AI, and machine learning in predictive maintenance
The combination of IoT, AI, and machine learning (ML) is making predictive maintenance smarter and more effective:
- IoT Sensors: Devices track real-time machine performance, ensuring continuous monitoring.
- AI & Machine Learning: Algorithms process sensor data, detect anomalies, and predict equipment failures.
- Cloud Computing: Scalable infrastructure stores and analyzes large datasets without latency issues.
A PwC study found that predictive maintenance using IoT and AI reduces maintenance costs by up to 40% and lowers downtime by up to 50%.
Data collection: leveraging real-time monitoring sensors
Industries leverage real-time condition monitoring to assess equipment performance through:
- Vibration analysis (detects mechanical issues in rotating machinery)
- Thermal imaging (monitors overheating electrical components)
- Acoustic analysis (identifies irregular noise patterns in engines and motors)
- Oil analysis (measures lubricant quality to prevent machinery wear)
Companies like Rolls-Royce use IoT-enabled aircraft engine monitoring, allowing them to remotely track thousands of engine performance parameters and schedule maintenance only when necessary.
Predictive analytics: how AI algorithms detect anomalies and failures
Advanced AI techniques, including deep learning and predictive modeling, analyze historical and real-time machine data to:
- Identify hidden failure patterns
- Classify normal vs. abnormal machine behavior
- Predict remaining useful life (RUL) of equipment
For instance, GE Digital’s AI-powered predictive analytics have helped industrial plants reduce machine failures by 30%, improving safety and efficiency.
Cloud computing: managing large-scale predictive maintenance datasets
Cloud platforms are essential for handling massive predictive maintenance datasets, ensuring seamless scalability and real-time processing. Key advantages include:
- Cost-efficient data storage: AWS, Azure, and Google Cloud offer flexible, pay-as-you-go data storage options.
- High-speed processing: Cloud-based AI engines quickly process maintenance alerts and predictions.
- Secure, remote access: Businesses can access maintenance reports and alerts from anywhere.
According to Deloitte, 90% of predictive maintenance programs rely on cloud-based platforms to process and analyze real-time equipment data.
3. Industry applications: how predictive maintenance is revolutionizing key sectors
Predictive maintenance has become a game-changer across industries, enhancing efficiency, reducing operational costs, and preventing unexpected equipment failures. Businesses leveraging AI-driven analytics, IoT, and cloud computing are seeing higher asset reliability, reduced downtime, and improved safety. Let’s explore how predictive maintenance is making an impact in different industries.
Manufacturing: optimizing equipment efficiency and minimizing downtime
The manufacturing industry relies on heavy machinery that must operate continuously to maintain production efficiency. Predictive maintenance helps manufacturers:
- Reduce unplanned downtime by up to 50% through early fault detection (Source: McKinsey).
- Increase asset lifespan by optimizing maintenance schedules based on real-time data.
- Enhance operational efficiency, as AI-driven maintenance strategies allow manufacturers to focus on production rather than constant equipment repairs.
Example: BMW uses IoT sensors and AI-based predictive maintenance to track vehicle assembly line performance, reducing machine failures by 30%. (Source: BMW Group)
Energy & utilities: preventing equipment failures in power plants
The energy sector faces high operational risks due to aging infrastructure and fluctuating demand. AI-powered predictive maintenance enables:
- Real-time monitoring of turbines, transformers, and power grids to detect anomalies before breakdowns occur.
- Up to 40% reduction in maintenance costs, improving power plant efficiency and grid stability
- Lower operational risks by preventing failures in critical energy infrastructure.
Example: General Electric (GE) employs AI-powered predictive maintenance for its power plants, reducing downtime by 30% and improving operational efficiency.
Automotive: reducing vehicle maintenance costs with AI diagnostics
Predictive maintenance in the automotive industry is transforming fleet management and personal vehicle maintenance by:
- Detecting potential failures in engines, brakes, and fuel systems before they cause breakdowns.
- Reducing vehicle maintenance costs by 25%, making fleet management more cost-effective.
- Extending vehicle lifespan through predictive diagnostics, reducing the need for frequent servicing.
Example: Tesla’s AI-based predictive maintenance allows vehicles to self-diagnose potential failures and alert owners before critical malfunctions. This has led to a 20% reduction in repair costs. (Source: Tesla)
Healthcare: improving medical equipment reliability and patient safety
The healthcare sector depends on high-precision medical devices that must function flawlessly. Predictive maintenance enhances:
- Early detection of equipment failures in MRI machines, ventilators, and patient monitors.
- Improved patient outcomes, as hospitals reduce the risk of machine-related failures.
- 25% lower maintenance costs for healthcare providers, leading to better resource allocation (Source: Frost & Sullivan).
Example: Siemens Healthineers uses AI-driven predictive maintenance for hospital imaging equipment, reducing breakdown rates by 30% and improving patient care.
Logistics & supply chain: ensuring smooth operations with AI-powered maintenance
Predictive maintenance is revolutionizing logistics and supply chain management by:
- Reducing unexpected vehicle breakdowns, ensuring uninterrupted delivery schedules.
- Improving warehouse equipment performance, such as conveyor belts, forklifts, and automated storage systems.
- Cutting supply chain maintenance costs by 20% while improving delivery accuracy
Example: Amazon’s AI-driven predictive maintenance optimizes warehouse robotics and delivery fleets, reducing maintenance costs by 30% and improving overall logistics efficiency.
Aviation: enhancing aircraft maintenance and passenger safety
In the aviation sector, predictive maintenance is critical for safety and efficiency. AI-powered analytics help:
- Detects potential aircraft component failures before takeoff, reducing in-flight risks.
- Improve aircraft turnaround time, ensuring flights remain on schedule.
- Save airlines up to $3 billion annually by preventing unplanned maintenance (Source: IATA).
Example: Boeing uses AI-based predictive maintenance to analyze real-time aircraft sensor data, reducing maintenance delays by 35%. (Source: Boeing)
5. Industry applications of predictive maintenance analytics
Predictive maintenance analytics is revolutionizing multiple industries by preventing equipment failures, reducing operational costs, and increasing productivity. From manufacturing and energy to healthcare and logistics, businesses are leveraging AI, IoT, and cloud computing to optimize asset performance and maximize uptime.
Manufacturing: minimizing downtime and optimizing production
Manufacturing companies depend on continuous production cycles, and equipment failures can result in significant financial losses. Predictive maintenance:
- Reduces machine downtime by up to 50%, leading to higher operational efficiency (Source: McKinsey).
- Lowers maintenance costs by 25-30% by predicting failures before they occur.
- Improves equipment lifespan by 20%, ensuring long-term asset reliability.
Example: General Motors (GM) uses AI-driven predictive maintenance to monitor factory machinery, reducing unexpected failures and increasing productivity.
Energy and utilities: ensuring grid stability and reducing failures
The energy sector relies on predictive maintenance to prevent power outages and optimize asset performance:
- Reduces transformer failures by 75%, ensuring uninterrupted power supply.
- Lowers maintenance costs by 40% through predictive analytics (Source: Deloitte).
- Improves efficiency of wind turbines by 15%, maximizing renewable energy production.
Example: Siemens Energy uses IoT and predictive analytics to monitor power grids, reducing downtime and improving energy distribution.
Healthcare: improving medical equipment reliability
Predictive maintenance plays a critical role in healthcare, ensuring that medical devices remain operational to support patient care. AI-driven maintenance:
- Prevents 80% of unexpected medical device failures, ensuring uninterrupted patient treatment (Source: GE Healthcare).
- Reduces equipment repair costs by 25%, allowing hospitals to allocate funds more efficiently.
- Ensures MRI and CT scanners operate at peak efficiency, minimizing diagnostic delays.
Example: Philips Healthcare leverages predictive analytics for medical imaging systems, reducing downtime and improving patient care.
Logistics and transportation: optimizing fleet management
Fleet operators use predictive maintenance to monitor vehicle health, reduce breakdowns, and enhance safety:
- Reduces fleet maintenance costs by 30%, optimizing vehicle repair schedules (Source: IBM).
- Increases vehicle uptime by 25%, ensuring uninterrupted deliveries and supply chain efficiency.
- Decreases fuel consumption by 10%, improving sustainability in logistics.
Example: UPS uses IoT-enabled predictive maintenance to track vehicle performance, reducing breakdowns and optimizing fleet management. (Source: UPS)
Aviation: preventing flight delays and ensuring passenger safety
Airlines rely on predictive maintenance to reduce aircraft downtime, enhance safety, and optimize maintenance schedules:
- Cuts aircraft maintenance costs by 20-30%, improving airline profitability.
- Reduces flight delays caused by technical issues by 35%, enhancing passenger experience.
- Extends aircraft component lifespan by 25%, ensuring long-term reliability.
Example: Lufthansa Technik uses AI-powered predictive analytics to monitor aircraft engine performance, reducing failures and improving operational efficiency.
6. Future trends in predictive maintenance analytics
Predictive maintenance analytics is evolving rapidly, with AI, machine learning, and IoT driving innovations that will redefine industrial efficiency. As organizations invest in smart maintenance strategies, emerging trends indicate a shift towards real-time analytics, automated decision-making, and AI-powered forecasting.
AI-powered predictive analytics: from reactive to proactive maintenance
- AI is transforming predictive maintenance from rule-based monitoring to self-learning models that improve over time.
- Machine learning algorithms now predict failures with 95% accuracy, reducing false alarms and unnecessary interventions.
- AI-driven automation reduces maintenance costs by 50% while boosting overall asset efficiency.
Example: IBM’s Watson AI analyzes real-time data from factory sensors to predict failures before they happen, reducing unplanned downtime. (Source: IBM)
Digital twins: simulating equipment behavior for enhanced accuracy
- Digital twin technology creates virtual replicas of physical assets, allowing businesses to simulate, analyze, and optimize maintenance strategies in real time.
- 77% of manufacturers are adopting digital twins to enhance predictive maintenance strategies.
- Predictive models built on digital twins improve maintenance scheduling accuracy by 30%, reducing unnecessary downtime.
Example: Siemens Energy employs digital twin technology to optimize turbine performance, reducing breakdowns and improving efficiency.
IoT-driven real-time monitoring: reducing downtime with edge analytics
- IoT-connected sensors continuously track asset health, detecting subtle performance changes before failure occurs.
- Predictive maintenance using IoT reduces downtime by 45% compared to traditional maintenance strategies.
- Edge computing accelerates predictive insights, reducing latency and enabling faster decision-making.
Example: GE Aviation integrates IoT-powered predictive analytics to monitor aircraft engine performance in real time, reducing maintenance costs.
Predictive maintenance meets blockchain: ensuring data security & transparency
- Blockchain enhances predictive maintenance by securing machine data, preventing fraud, and ensuring transparency in maintenance logs.
- Over 65% of enterprises are exploring blockchain integration for predictive maintenance to reduce data tampering risks.
- Smart contracts in blockchain automate maintenance scheduling, ensuring adherence to compliance regulations.
Example: Maersk employs blockchain-powered predictive maintenance to track real-time shipping container conditions, improving fleet reliability.
The shift towards prescriptive maintenance: the next frontier
- Prescriptive maintenance goes beyond prediction—it suggests optimal actions to prevent failures, optimizing resources in real time.
- Organizations that implement prescriptive maintenance experience 10-20% greater operational efficiency than predictive maintenance users alone.
- AI-driven prescriptive analytics reduces maintenance-related downtime by 60%, maximizing equipment lifespan.
Example: Airbus uses prescriptive maintenance to forecast and automatically adjust maintenance schedules, reducing disruptions in airline operations. (Source: Airbus)
7. The future of predictive maintenance is now
Predictive maintenance analytics is no longer a futuristic concept—it is the present and future of efficient, cost-effective, and data-driven asset management. With AI, IoT, digital twins, and blockchain revolutionizing industrial maintenance, organizations that embrace these technologies gain a significant competitive advantage.
Why predictive maintenance is a game changer
- Predictive maintenance reduces unplanned downtime by up to 50%, minimizing revenue losses.
- AI-powered analytics increases equipment lifespan by 20-40%, reducing replacement costs.
- IoT-driven real-time monitoring improves maintenance efficiency by 45%, enabling proactive interventions.
- Prescriptive maintenance enhances operational performance by 20%, ensuring continuous uptime.
By integrating AI-powered predictive maintenance analytics, organizations can transition from reactive and scheduled maintenance to intelligent, real-time, and cost-saving strategies.
Adopting predictive maintenance with Applify’s AI & cloud services
For businesses looking to leverage AI-driven predictive maintenance, AI & Data Services provide advanced machine learning solutions, while Cloud Services offer scalable IoT and cloud-based predictive maintenance platforms.
The future belongs to businesses that act now
Organizations that invest in predictive analytics today will reap the benefits of lower costs, higher efficiency, and greater asset longevity tomorrow. The time to transition from traditional maintenance methods to AI-powered predictive strategies is now—because waiting means risking downtime, inefficiency, and higher operational costs.

Surbhi Goyal is the Vice President of Product with expertise in AI-driven solutions for small and medium businesses (SMBs). She specializes in helping organizations adopt scalable, cloud-based AI technologies to improve efficiency and deliver better customer experiences. Surbhi’s practical approach ensures businesses can leverage AI effectively to drive growth and innovation.
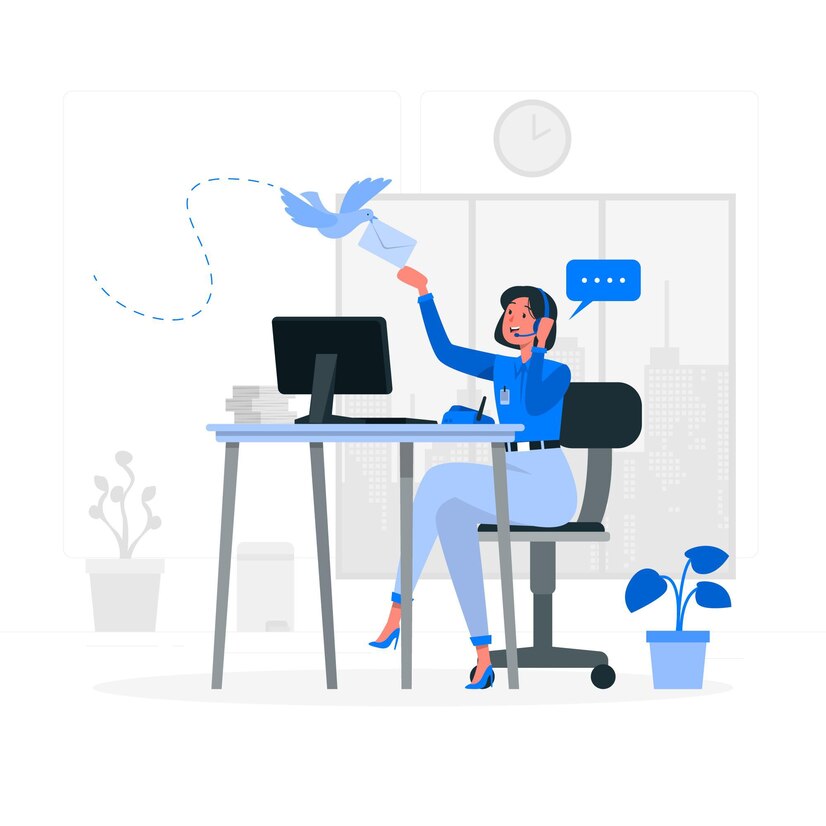
Have questions or need assistance? Fill out the form below, and we’ll get back to you as soon as possible.