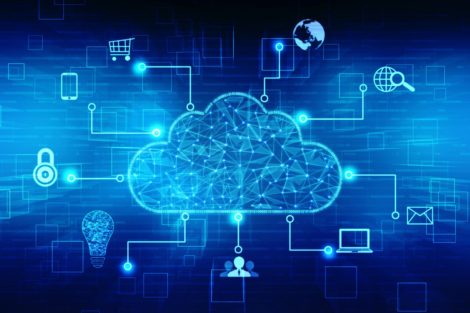
1. Moving beyond traditional cloud computing
The evolution of data processing from centralized to decentralized models
For decades, data processing followed a centralized approach, where information was gathered, processed, and stored in large, remote data centers. This model worked well when businesses dealt with limited volumes of structured data. However, with the rise of Internet of Things (IoT) devices, real-time analytics, and AI-powered applications, organizations now require faster, decentralized solutions.
Traditional cloud computing—while efficient for many applications—struggles with the latency, bandwidth, and security risks associated with real-time data processing. Edge computing emerged as a solution to these challenges, bringing computation and data storage closer to the source rather than relying solely on distant cloud servers.
According to Accenture's Edge Computing Index, by 2025, 75% of enterprise data will be processed at the edge, reducing latency and bandwidth costs while improving security (Accenture).
How edge computing bridges the gap between cloud and on-premise systems
- Cloud computing remains cost-effective and scalable, but it struggles with low-latency, real-time applications (e.g., autonomous vehicles, smart factories).
- On-premise computing ensures data control and security, but lacks scalability and flexibility.
- Edge computing acts as a hybrid model, enabling organizations to process critical data locally while leveraging the cloud for large-scale analytics, AI training, and long-term storage.
This edge-cloud synergy ensures that businesses optimize cost, speed, and security in their digital transformation strategies.
The role of 5G, IoT, and AI in accelerating edge adoption
Three key technological drivers are fueling the widespread adoption of edge computing:
- 5G Networks: Ultra-fast, low-latency 5G connectivity enables seamless real-time processing at the edge.
- IoT Devices: The explosion of connected devices (expected to reach 29.4 billion globally by 2030 according to Statista (source)) generates massive amounts of real-time data.
- AI & Machine Learning: AI models at the edge allow businesses to make intelligent, instant decisions without sending data back to centralized cloud servers.
Real-world applications of edge computing in various industries
Edge computing is already transforming multiple industries by enhancing speed, efficiency, and security in data-driven operations:
- Healthcare: AI-assisted diagnostics and remote patient monitoring
- Retail: Smart inventory management and personalized customer experiences
- Manufacturing: Predictive maintenance and robotics automation
- Finance: Real-time fraud detection and high-frequency trading
- Transportation: Self-driving cars and intelligent traffic management
Organizations investing in edge computing solutions can achieve up to 40% lower cloud costs and improve operational efficiencies by 25% or more (IBM).
2. Breaking down edge computing: how it works and why it matters
How edge computing processes data closer to the source
Unlike traditional cloud computing, where data travels to centralized servers for processing, edge computing pushes computation closer to where data is generated—whether it's an IoT sensor, a connected vehicle, or a smart factory machine.
This localized processing model follows three key steps:
- Data Generation – Devices, sensors, and machines collect vast amounts of real-time data.
- Edge Processing – Instead of sending all data to the cloud, edge servers or on-device AI models filter, analyze, and process critical information locally.
- Cloud Integration – Only necessary insights and processed data are transmitted to cloud servers for long-term storage, analytics, or deeper AI model training.
By reducing data transmission requirements, edge computing significantly improves speed, security, and cost efficiency.
Why latency, bandwidth, and security make edge computing a game-changer
- Latency Reduction: Traditional cloud architectures introduce network delays due to physical distances between devices and data centers. Edge computing ensures real-time responses, essential for autonomous vehicles, industrial automation, and telemedicine applications.
- Bandwidth Optimization: Streaming vast amounts of raw data to the cloud is expensive and inefficient. Edge computing reduces bandwidth costs by filtering and compressing data before transmission.
- Enhanced Security & Privacy: Since sensitive data remains within local edge networks, businesses minimize exposure to cyber threats and comply with strict data sovereignty regulations.
According to Cloudflare, businesses leveraging edge computing experience up to a 60% improvement in response time and a 30% reduction in cloud storage costs (Cloudflare).
The role of AI and machine learning in edge computing
AI is not only transforming the cloud but also redefining edge computing capabilities. With the rise of on-device AI models and federated learning, businesses can now deploy:
- AI-powered security cameras that detect threats without sending footage to the cloud.
- Predictive maintenance algorithms in manufacturing to anticipate machine failures in real time.
- AI-driven retail experiences, such as personalized in-store recommendations based on customer behavior analysis.
According to Accenture, edge AI adoption is expected to increase by 55% in the next three years, transforming industries reliant on real-time decision-making (Accenture).
3. Industries leading the edge computing revolution
1. Smart manufacturing: predictive maintenance and automation
Edge computing is revolutionizing the Industrial Internet of Things (IIoT) by enabling real-time predictive maintenance, reducing operational downtime, and increasing efficiency.
- Predictive maintenance: AI-powered edge sensors detect early signs of equipment failure, preventing costly breakdowns.
- Automated quality control: Cameras and IoT sensors process defect detection instantly on the factory floor, improving production efficiency.
- Low-latency robotics: Edge-powered robots adjust manufacturing processes in real time without needing cloud access.
According to McKinsey, manufacturers implementing edge computing reduce machine downtime by up to 50%, saving millions in lost productivity (McKinsey).
2. Healthcare: real-time diagnostics and remote patient monitoring
The healthcare industry is leveraging edge computing to enhance diagnostic speed, patient monitoring, and telemedicine.
- Medical imaging analysis: AI algorithms process X-rays, MRIs, and CT scans on-premises to detect diseases faster and more accurately.
- Wearable health monitoring: Smart devices process heart rate, glucose levels, and oxygen saturation in real time, reducing data lag and enhancing patient care.
- Telemedicine consultations: Edge-powered video calls reduce latency, providing seamless virtual healthcare experiences.
A study by Intel Healthcare found that real-time edge AI diagnostics improve patient response time by up to 30%, significantly boosting treatment outcomes (Intel).
3. Autonomous vehicles: instant decision-making on the road
Self-driving cars require millisecond-level processing to interpret road conditions, detect obstacles, and make decisions without depending on the cloud.
- AI-driven perception: Cameras, LiDAR, and radar sensors process driving conditions locally, ensuring instant reaction times.
- Vehicle-to-everything (V2X) communication: Cars interact with traffic lights, pedestrian crossings, and other vehicles via edge-enabled connectivity.
- Advanced driver assistance systems (ADAS): AI-powered alerts assist human drivers in real-time, reducing collision risks.
According to Gartner, edge computing will power 90% of new autonomous vehicle functionalities by 2026, making roadways safer and more efficient (Gartner).
4. Retail: hyper-personalized shopping experiences
Retailers are adopting edge computing to optimize in-store experiences, streamline supply chains, and improve customer engagement.
- Smart checkout systems: AI-enabled cashier-less checkout eliminates long queues.
- Personalized recommendations: Edge AI analyzes shopper behavior instantly, delivering tailored promotions in-store.
- Inventory management: Real-time monitoring prevents stock shortages, ensuring efficient supply chain operations.
According to Accenture, retailers using edge AI see a 25% increase in customer engagement and a 15% boost in revenue due to real-time personalized experiences (Accenture).
5. Finance: fraud detection and real-time transaction processing
Financial institutions are integrating edge AI for fraud prevention, high-frequency trading, and instant payments.
- Fraud detection: AI scans transactions in real time for suspicious activity, preventing fraud before it happens.
- Algorithmic trading: Edge computing allows firms to execute trades in milliseconds, ensuring competitive advantage.
- Secure contactless payments: Reduces transaction lag and improves mobile payment experiences.
A report by Forrester states that edge AI reduces false positives in fraud detection by 40%, improving financial security and reducing losses (Forrester).
6. Energy & smart cities: optimizing urban efficiency
Cities and energy companies are deploying edge computing to optimize traffic systems, manage energy grids, and enhance public safety.
- Smart traffic lights: AI-driven systems adjust traffic signals dynamically to reduce congestion.
- Grid automation: Edge AI optimizes electricity distribution, reducing energy waste.
- Public surveillance: Edge-powered CCTV cameras improve crime detection and response times.
A report by IDC found that smart cities adopting edge AI reduce energy consumption by 30%, enhancing sustainability efforts (IDC).
4. The role of AI and machine learning in edge computing
Edge computing and artificial intelligence (AI) are converging to create powerful, real-time data processing capabilities across industries. AI-powered edge devices bring machine learning (ML) models closer to the data source, reducing reliance on centralized cloud infrastructure.
1. AI at the edge: why it matters
Traditional AI applications rely on cloud-based processing, but AI at the edge offers several benefits:
- Ultra-low latency – AI models process data locally, enabling instant decision-making for time-sensitive applications like autonomous vehicles and industrial automation.
- Reduced bandwidth usage – Processing data on-device minimizes costly cloud data transfers, optimizing network efficiency.
- Enhanced privacy & security – Sensitive data remains on-premises rather than being transmitted to the cloud, mitigating cyber risks.
According to Gartner, by 2025, 75% of enterprise-generated data will be created and processed outside of centralized cloud data centers, reflecting the shift towards AI-driven edge computing (Gartner).
2. Machine learning models optimized for edge computing
Edge AI leverages specialized machine learning models designed for low-power, high-speed operations:
- Federated Learning: AI models train locally on edge devices, improving performance while maintaining data privacy.
- TinyML: Enables microcontroller-level AI processing, allowing energy-efficient AI applications in IoT devices.
- Pruned Neural Networks: Streamlined deep learning models require less computational power, making AI feasible on compact edge hardware.
A report by Accenture states that AI-powered edge solutions can increase operational efficiency by 30%, particularly in manufacturing and logistics (Accenture).
3. Real-world applications of ai-driven edge computing
Industries are rapidly integrating AI and ML into edge computing ecosystems to enhance automation and intelligence:
- Smart Surveillance: AI-powered edge cameras detect anomalies in real-time, improving security and reducing false alarms.
- Autonomous Retail: AI-driven self-checkout systems process customer purchases instantly, reducing friction in retail transactions.
- Predictive Maintenance: Machine learning models analyze sensor data locally to detect early signs of equipment failure, preventing costly downtime.
According to IDC, organizations deploying AI-powered edge computing solutions experience a 40% reduction in cloud processing costs while improving response times (IDC).
5. Key industries benefiting from edge computing
Edge computing is revolutionizing multiple industries by bringing data processing closer to the source, improving real-time analytics, and reducing latency. Organizations across sectors are leveraging edge infrastructure to optimize operations, enhance security, and enable faster decision-making.
1. Manufacturing: smart factories and automation
Edge computing is at the heart of Industry 4.0, enabling predictive maintenance, robotics, and automated quality control in manufacturing.
- Real-time monitoring – IoT sensors collect and analyze data at the machine level, preventing downtime and reducing operational costs.
- AI-driven automation – Robotic systems powered by edge AI optimize production lines, minimizing defects and waste.
- Enhanced worker safety – Edge-powered computer vision detects safety hazards instantly, preventing workplace accidents.
According to McKinsey, manufacturers using edge computing experience a 30-50% reduction in unplanned downtime, significantly improving productivity (McKinsey).
2. Healthcare: AI-powered diagnostics and patient monitoring
Edge computing is transforming healthcare and telemedicine by enabling real-time diagnostics, monitoring, and robotic-assisted procedures.
- Remote patient monitoring – Wearable devices process vital signs locally, alerting doctors about anomalies instantly.
- Medical imaging & diagnostics – AI-driven edge devices enhance X-ray and MRI analysis, reducing cloud dependency.
- Autonomous robotic surgery – Edge-powered surgical systems enable precise, real-time adjustments, improving patient outcomes.
A Deloitte study highlights that AI-driven edge healthcare solutions reduce hospital readmission rates by 25%, enhancing efficiency and patient care (Deloitte).
3. retail: enhancing customer experience with real-time analytics
Edge computing is transforming the retail industry by personalizing shopping experiences, streamlining checkout, and optimizing inventory management.
- AI-powered smart shelves – Edge sensors track real-time stock levels, reducing out-of-stock scenarios.
- Seamless checkout experiences – Edge-driven self-checkout systems eliminate long queues and enhance convenience.
- Personalized marketing – Edge AI analyzes customer behavior, delivering real-time targeted promotions.
Retailers using edge AI-driven customer insights have reported a 25% increase in conversion rates due to improved personalization strategies (Accenture).
4. Autonomous vehicles & transportation: real-time decision-making on the move
The automotive industry is leveraging edge computing for self-driving cars, fleet management, and traffic optimization.
- Real-time hazard detection – Edge AI processes data from cameras, LiDAR, and radar, ensuring quick collision avoidance.
- Smart traffic management – Edge-based analytics improve traffic flow, reducing congestion and fuel consumption.
- Fleet optimization – AI-driven predictive maintenance enhances vehicle longevity and efficiency.
According to Intel, autonomous vehicles generate 4TB of data per hour, making edge computing a necessity to process critical information in real-time (Intel).
5. Financial services: fraud detection and risk management
Financial institutions are harnessing edge computing for fraud detection, algorithmic trading, and secure transactions.
- AI-driven fraud detection – Edge analytics identify suspicious transactions instantly, reducing financial losses.
- Algorithmic trading – Low-latency edge computing accelerates trade execution, improving market efficiency.
- Personalized banking experiences – Real-time customer insights enhance service personalization and engagement.
Financial firms utilizing real-time edge fraud detection have reduced cyberattack response times by 45%, significantly improving security (IBM).
6. Telecommunications: 5g and edge computing synergy
The 5G revolution is accelerating the adoption of edge computing, enhancing network efficiency and enabling ultra-fast connectivity.
- Low-latency content delivery – Edge nodes cache and process data locally, improving video streaming and gaming experiences.
- IoT network optimization – Telecom providers use edge AI to manage millions of connected devices efficiently.
- Edge-powered smart cities – Traffic control, energy grids, and public safety solutions operate in real-time using 5G-enabled edge networks.
According to Gartner, by 2025, 60% of telecom infrastructure will rely on edge computing, enhancing global connectivity (Gartner).
6. The intersection of edge computing and artificial intelligence
Edge computing and artificial intelligence (AI) are forming a powerful combination, enabling faster data processing, real-time decision-making, and automation at the source. This intersection is transforming industries by minimizing cloud dependency, reducing latency, and improving security.
1. Why AI needs edge computing
AI models require massive data processing capabilities, often relying on cloud computing. However, transmitting large volumes of data to the cloud introduces latency and security risks. This is where edge computing steps in to enhance AI's efficiency by processing data locally before it reaches the cloud.
Key benefits include:
- Reduced latency – AI models can analyze and act on data in real-time, critical for applications like autonomous vehicles, industrial automation, and healthcare monitoring.
- Improved security – Edge computing minimizes the need to send sensitive data to the cloud, reducing exposure to cyber threats.
- Lower bandwidth costs – AI workloads are distributed across edge devices, preventing excessive cloud bandwidth usage.
According to IDC, by 2025, 50% of AI workloads will be deployed at the edge to improve speed and security (IDC).
2. Edge AI: real-time intelligence at the source
Edge AI refers to running AI models directly on edge devices such as IoT sensors, cameras, and industrial equipment. These models make split-second decisions without relying on centralized cloud infrastructure.
Examples of edge AI applications:
- Smart security cameras – AI-powered cameras analyze video feeds in real-time to detect suspicious activities without cloud processing delays.
- Predictive maintenance – AI models deployed at the edge monitor machinery and equipment health, predicting failures before they occur.
- Voice and facial recognition – AI on smartphones and IoT devices ensures instant authentication without cloud dependencies.
A Gartner report states that by 2027, 75% of enterprise-generated data will be processed at the edge, demonstrating the growing adoption of edge AI (Gartner).
3. AI-powered edge computing in action
Leading cloud providers, including AWS, Google Cloud, and Microsoft Azure, are integrating AI with edge computing to enhance efficiency, scalability, and security.
- AWS IoT Greengrass – Runs machine learning inference on edge devices, optimizing IoT automation.
- Google Cloud Edge TPU – Enables high-performance AI inference directly on edge hardware.
- Microsoft Azure Percept – Delivers pre-built AI models for edge devices in industrial and retail applications.
Industries leveraging AI-powered edge computing include:
- Healthcare – AI-based remote patient monitoring systems reduce hospital visits while enhancing proactive care.
- Retail – Edge AI-driven inventory management systems prevent stock shortages and optimize pricing strategies.
- Logistics – AI-powered autonomous delivery drones use edge processing for route optimization.
A study by Accenture highlights that businesses integrating AI with edge computing experience a 35% boost in operational efficiency, accelerating digital transformation (Accenture).
7. Industries transforming with edge computing
Edge computing is redefining industries by enhancing efficiency, lowering costs, and enabling real-time insights. With global edge computing investments expected to reach $250 billion by 2025 (IDC), businesses across sectors are leveraging this technology to revolutionize operations.
1. Healthcare: real-time patient monitoring and diagnostics
Edge computing is empowering healthcare providers with real-time patient data, improving diagnostics, treatment plans, and emergency response.
Key applications:
- Remote patient monitoring – IoT-enabled medical devices collect and analyze patient vitals at the edge, reducing hospital visits and improving home care.
- AI-assisted diagnostics – Edge AI enhances MRI and X-ray analysis, detecting abnormalities with greater accuracy and speed.
- Telemedicine advancements – Low-latency video consultations powered by edge computing improve accessibility to healthcare professionals.
2. Manufacturing: predictive maintenance and automation
Smart factories powered by edge computing enable real-time automation, predictive maintenance, and streamlined production workflows.
Key applications:
- Predictive maintenance – Sensors analyze machine performance at the edge, predicting failures before they occur, reducing downtime.
- Quality control – AI-driven edge computing detects defects in real-time during assembly, ensuring high product standards.
- Automated robotics – Edge-powered robotic arms in production lines operate autonomously with ultra-low latency.
3. Retail: personalized shopping experiences
Edge computing is reshaping the retail landscape by improving customer experience, optimizing inventory, and reducing operational costs.
Key applications:
- Smart inventory management – Sensors in warehouses track stock levels and prevent shortages in real-time.
- Frictionless checkout systems – AI-powered checkout counters in stores process payments instantly without long queues.
- Hyper-personalized shopping – Edge AI analyzes in-store shopper behavior, delivering customized promotions and product recommendations.
4. Transportation and logistics: real-time fleet management
Edge computing optimizes logistics operations, improves fleet efficiency, and enhances supply chain visibility.
Key applications:
- Autonomous vehicle navigation – Self-driving cars process real-time road data at the edge for immediate decision-making.
- Fleet monitoring – AI-powered edge sensors track vehicle performance, predicting maintenance needs.
- Route optimization – Real-time traffic data helps logistics companies minimize delivery times.
5. Financial services: fraud detection and security
Edge computing enhances banking security and fraud detection by analyzing transactions in real-time.
Key applications:
- AI-powered fraud detection – Suspicious transactions are flagged instantly, preventing fraud before it happens.
- Biometric authentication – Facial and fingerprint recognition processes identity verification on edge devices for faster transactions.
- ATM security enhancements – Real-time monitoring prevents card skimming and cyber threats at ATMs.
6. Energy and utilities: smart grid optimization
Edge computing is powering the future of energy management by making electricity grids more intelligent, resilient, and sustainable.
Key applications:
- Smart grid monitoring – Edge-powered IoT sensors track energy usage and distribution, preventing blackouts.
- Renewable energy optimization – AI at the edge helps wind and solar farms adjust output based on weather conditions.
- Industrial energy efficiency – Edge-based analytics optimize power consumption in factories and plants.
Edge computing is the future of industry 4.0
From healthcare to finance, edge computing is transforming industries by enabling real-time decision-making, reducing costs, and improving security. As more organizations shift towards a decentralized computing model, edge technology will continue to drive innovation, efficiency, and business growth.
For businesses looking to leverage edge computing for AI-driven analytics, cloud solutions, or custom software development, explore how Cloud Solutions can help your enterprise stay ahead of the curve.

Surbhi Goyal is the Vice President of Product with expertise in AI-driven solutions for small and medium businesses (SMBs). She specializes in helping organizations adopt scalable, cloud-based AI technologies to improve efficiency and deliver better customer experiences. Surbhi’s practical approach ensures businesses can leverage AI effectively to drive growth and innovation.
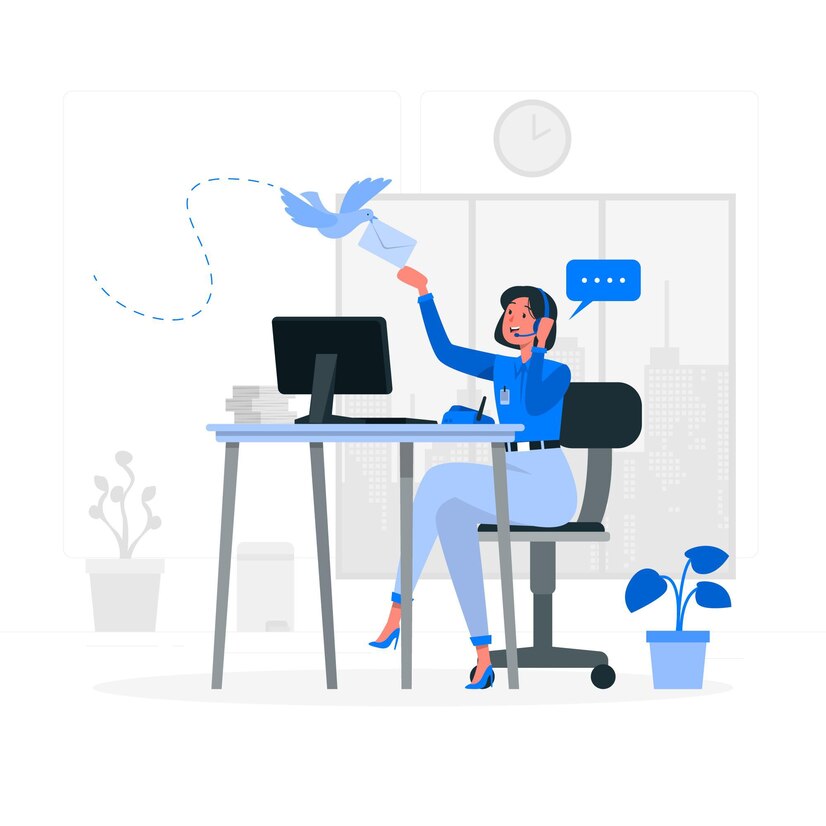
Have questions or need assistance? Fill out the form below, and we’ll get back to you as soon as possible.