Unexpected equipment failures cost businesses billions every year. According to Gartner, companies experience an average downtime cost of $260,000 per hour. With increasing complexity in industrial machinery, traditional maintenance approaches are no longer sufficient.
Data analytics for predictive maintenance is changing the game by using AI, machine learning, and iot sensors to predict failures before they happen. This proactive approach is reducing downtime, cutting maintenance costs, and improving asset reliability across industries.
In this article, we’ll explore how predictive maintenance works, its real-world applications, the role of ai data service, and emerging trends that will shape the future of asset management.
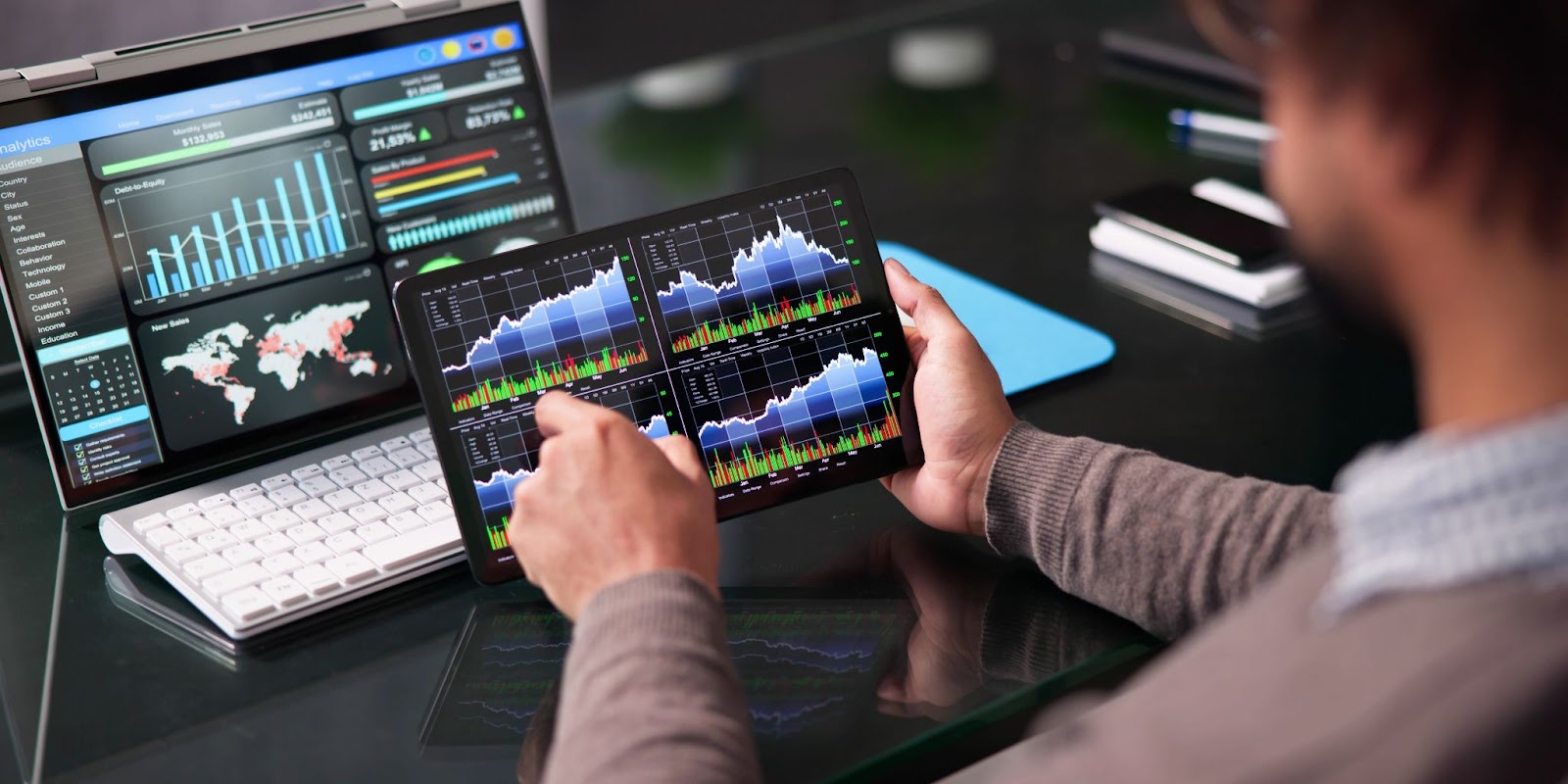
What is predictive maintenance?
Predictive maintenance (PdM) is a proactive maintenance strategy that uses data analytics, machine learning, and IoT sensors to detect early signs of equipment failure. Unlike reactive maintenance (fixing equipment after failure) or preventive maintenance (servicing equipment at scheduled intervals), predictive maintenance optimizes maintenance schedules based on real-time data.
How predictive maintenance works
- Data collection – sensors collect data from machines, including temperature, vibration, pressure, and operational status.
- Data storage – raw sensor data is stored in a data lake, allowing for real-time and historical analysis.
- Data processing & analytics – machine learning models analyze trends and patterns to detect early warning signs of failure.
- Predictive insights – AI-powered analytics generate alerts, helping maintenance teams take action before breakdowns occur.
Rethinking maintenance: from reactive to predictive
The traditional approach is broken
For decades, businesses have relied on reactive and preventive maintenance:
- Reactive maintenance – fix equipment only after it fails (costly and disruptive).
- Preventive maintenance – perform maintenance on a fixed schedule (often unnecessary and expensive).
Both methods lead to inefficiencies, high costs, and unexpected breakdowns.
Why predictive maintenance is the future
Predictive maintenance (PdM) uses real-time data and AI-driven analytics to:
- detect early signs of equipment failure
- predict the optimal time for maintenance
- minimize downtime and reduce unnecessary repairs
Companies adopting predictive maintenance see a 30-50% reduction in downtime and a 40% decrease in maintenance costs.
How predictive maintenance analytics works
1. Data collection from IoT sensors
Industrial sensors collect real-time data on:
- Temperature & vibration – detect overheating and misalignment
- Pressure & flow rates – monitor fluid dynamics in pipelines
- Electrical signals – analyze power fluctuations in machinery
2. Data storage & integration
Data lakes store raw machine data from multiple sources, enabling businesses to:
- Integrate real-time and historical data for better predictions
- Process high-volume data efficiently
- Use data lakes consulting for seamless implementation
3. AI & machine learning models analyze data
- anomaly detection – ai identifies deviations from normal operating conditions
- predictive algorithms – machine learning models predict failure probabilities
- automated recommendations – ai suggests maintenance actions before failures occur
4. Predictive insights & automated maintenance
Maintenance teams receive real-time alerts and AI-generated work orders to fix issues before they escalate.

Key benefits of predictive maintenance analytics
1. Real-time failure prediction
By analyzing vast amounts of operational data, predictive maintenance provides real-time alerts about potential failures, preventing unexpected breakdowns.
2. Optimizing maintenance costs
businesses often spend too much on unnecessary maintenance. AI-driven insights help optimize maintenance schedules, reducing costs by 40%.
3. Extending asset lifespan
Predictive maintenance identifies wear and tear before it leads to major failures, significantly increasing asset lifespan.
4. Enhancing workplace safety
Equipment malfunctions are a major safety risk. Predictive analytics ensures safer working conditions by preventing failures before they happen.
5. Increasing operational efficiency
According to Accenture, predictive maintenance improves overall equipment effectiveness (OEE) by up to 25% (accenture, 2023).
Industry applications: where predictive maintenance is making an impact
Manufacturing
- AI-powered artificial intelligence in manufacturing detects faulty machinery before production stops.
- Predictive maintenance in robotics reduces factory downtime.
Healthcare
- AI in healthcare ensures the reliability of critical medical devices.
- Hospitals use predictive analytics to prevent MRI and CT scanner failures.
Transportation & logistics
- AI tracks engine health in fleet vehicles, airplanes, and trains to prevent failures.
- Airlines save millions per year by predicting turbine failures before they happen.
Energy & utilities
- Power plants monitor turbines and transformers to prevent outages.
- Renewable energy companies use ai to optimize wind turbine maintenance.
AI and data lakes: the foundation of predictive maintenance
Why AI is a game-changer
By integrating AI data service into predictive maintenance, businesses can:
- automate decision-making with intelligent agents
- improve accuracy using natural language processing in artificial intelligence
- integrate ai chatbots for real-time diagnostics (enterprise AI chatbot)
How data lakes power predictive maintenance
Data lakes store and process massive amounts of machine data, enabling businesses to:
- analyze real-time historical data for accurate predictions
- train advanced AI models for smarter failure detection
- seamlessly scale predictive maintenance solutions
Data lakes consulting can help companies set up the right architecture for AI-driven predictive maintenance.
Emerging trends shaping predictive maintenance
1. Self-healing machines
AI-driven systems are evolving to self-correct minor faults automatically, reducing human intervention.
2. Digital twins
Businesses are using digital replicas of machinery to simulate real-world performance and predict failures with greater accuracy.
3. Predictive maintenance-as-a-service
Cloud-based platforms are making ai-powered predictive maintenance more accessible through cloud services trends.
4. AI-powered voice assistants for maintenance teams
Technicians can use voice-enabled AI assistants to get real-time diagnostics and repair instructions, powered by intelligent agents.
Why predictive maintenance is a must-have
Predictive maintenance analytics is no longer optional—it's a business necessity. companies that fail to adopt ai-powered predictive maintenance risk:
- costly downtime
- excessive maintenance expenses
- reduced equipment lifespan
On the other hand, businesses leveraging AI and data lakes can:
- cut downtime by 50%
- reduce maintenance costs by 40%
- improve operational efficiency and asset reliability
Ready to transform your maintenance strategy?
Get in touch with our AI experts to implement cutting-edge predictive maintenance solutions for your business!