1. The new battleground for enterprise data
The rise of AI, cloud computing, and real-time analytics has dramatically altered how businesses perceive data storage and processing. Organizations are no longer just collecting data—they need instant access to insights to remain competitive. However, with data growing at an unprecedented rate, legacy storage systems are struggling to keep up.

Why businesses today are drowning in data but starving for insights
The amount of data generated worldwide is projected to reach 180 zettabytes by 2025, up from 79 zettabytes in 2021. However, a staggering 73% of enterprise data remains unused for analytics. The reason? Many organizations lack the right data storage and retrieval strategies.
- Traditional databases and on-premise storage solutions weren’t designed for AI-powered analytics.
- Business leaders struggle with data silos, governance challenges, and performance bottlenecks when extracting insights from disparate systems.
- Without a scalable, cloud-native approach, businesses risk losing the competitive edge to those that leverage real-time, AI-driven decision-making.
The outdated ‘one-size-fits-all’ approach to data storage
For years, enterprises relied on relational databases and structured data warehouses for analytics and reporting. But today, data comes in multiple formats—structured, semi-structured, and unstructured—and needs to be processed at scale.
- 80% of enterprise data is unstructured, coming from sources like IoT devices, social media, video, and emails.
- Traditional data warehouses struggle to handle unstructured and streaming data, limiting an organization’s ability to leverage AI and real-time analytics.
- A more flexible solution—such as a data lake, data warehouse, or a hybrid approach (Lakehouse model)—is essential for modern businesses.
How AI, cloud computing, and real-time analytics are redefining data storage needs
As AI adoption accelerates, businesses are rethinking their data architectures. AI-driven organizations need storage solutions that ingest, process, and analyze vast volumes of structured and unstructured data seamlessly.
- 87% of enterprises believe AI will drive revenue growth, but lack of AI-ready data infrastructure is a major barrier.
- Cloud-based AI and analytics solutions are growing at a 40% CAGR, signaling a shift toward scalable, serverless computing for data processing.
- Enterprises adopting cloud-based data lakes and warehouses see a 2x increase in analytics efficiency and a 50% reduction in operational costs.
With the rise of AI-driven data strategies, businesses can no longer afford fragmented and outdated data storage models. This leads to the next crucial question:
How do data lakes and data warehouses fit into the modern enterprise data ecosystem?Why data lakes and data warehouses were createdAs enterprises faced exponential data growth, traditional storage models became insufficient. The demand for scalable, AI-ready architectures led to two dominant solutions—data lakes and data warehouses. While both serve as centralized data repositories, their purposes, architectures, and efficiencies differ significantly.

2. Why data warehouses emerged: the structured data era
Data warehouses were designed when businesses relied primarily on structured data—organized, tabular information stored in relational databases. Companies needed a high-performance system for reporting and analytics, leading to the creation of data warehouses in the 1990s.
- Primary use case: Business intelligence (BI), reporting, and structured analytics.
- Key industries: Banking, finance, retail, healthcare—where structured, transactional data dominates.
- Core strength: Optimized for pre-defined, structured queries to generate fast insights.
According to Gartner, 75% of enterprises still use data warehouses for traditional analytics, but they struggle with modern AI workloads due to scalability limitations.
Why data lakes emerged: the unstructured data explosion
As businesses adopted IoT, social media, machine logs, and multimedia data, the need for unstructured data storage surged. Data lakes, popularized in the 2010s, allowed companies to store raw, unprocessed data from multiple sources—structured and unstructured—without rigid schema constraints.
- Primary use case: AI, machine learning (ML), big data analytics, and real-time insights.
- Key industries: E-commerce, manufacturing, healthcare, and smart cities—where sensor data, video, and logs dominate.
- Core strength: Stores massive amounts of raw, diverse data for AI-driven processing.
A McKinsey study found that organizations leveraging data lakes improve AI adoption by 30%, enabling better decision-making and automation.
Key differences: when to choose a data lake vs. data warehouse

Many businesses struggle to choose between data lakes vs. data warehouses, but forward-thinking organizations combine both to create a hybrid model—the data lakehouse—which blends the flexibility of lakes with the structure of warehouses.
3. AI meets data: the role of artificial intelligence in shaping data storage
The rise of artificial intelligence (AI) and machine learning (ML) has transformed how businesses store, manage, and extract value from data. Traditional data architectures—primarily data warehouses—were built for structured queries and historical analysis, whereas modern AI applications demand scalability, real-time insights, and diverse data processing capabilities. This shift is driving massive investments in data lakes, which can store raw, unstructured, and semi-structured data, making it ideal for AI-driven innovation.
How AI is driving the evolution of data storage
According to Gartner, by 2025, 50% of enterprises will adopt AI-driven data lakes for advanced analytics, a significant shift from traditional BI-focused data warehouses. This evolution is happening due to several AI-related factors:
- Unstructured data explosion: AI models require massive volumes of diverse data—text, images, videos, IoT sensor data, and logs—which data lakes are optimized to store.
- Real-time analytics demand: AI applications, such as predictive maintenance and fraud detection, require real-time processing that traditional data warehouses struggle to support.
- Self-learning data pipelines: AI-enhanced data lakes can automate schema evolution, data categorization, and anomaly detection, improving efficiency.
AI-powered automation in data lakes vs. data warehouses
AI and automation have fundamentally changed how data lakes and data warehouses operate:

How businesses leverage AI-driven data lakes
- Predictive analytics for manufacturing
- AI-powered data lakes help manufacturers analyze IoT sensor data to predict machine failures before they occur, reducing downtime and maintenance costs.
- Companies like GE and Siemens leverage AI to process vast amounts of industrial data, enabling 30% more efficient operations.
- Personalized marketing in e-commerce
- AI models process customer behavior data from multiple channels, including web activity, social media, and purchase history, to generate hyper-personalized recommendations.
- Amazon’s recommendation engine, powered by AI-driven data lakes, contributes 35% of its total revenue.
- Financial fraud detection
- AI-driven data lakes store and analyze real-time transaction data to detect fraudulent activities with 99% accuracy.
- Leading banks use AWS Lake Formation to enable secure, AI-driven fraud analytics.
4. Data lake, data warehouse, or data lakehouse—what’s the right choice?
As businesses adopt more AI-powered analytics and real-time decision-making, the debate between data lakes and data warehouses has evolved. A new hybrid solution—the data lakehouse—has emerged to bridge the gap between the two. The key question for organizations today is: Which data architecture best fits their needs?
Understanding the strengths and limitations
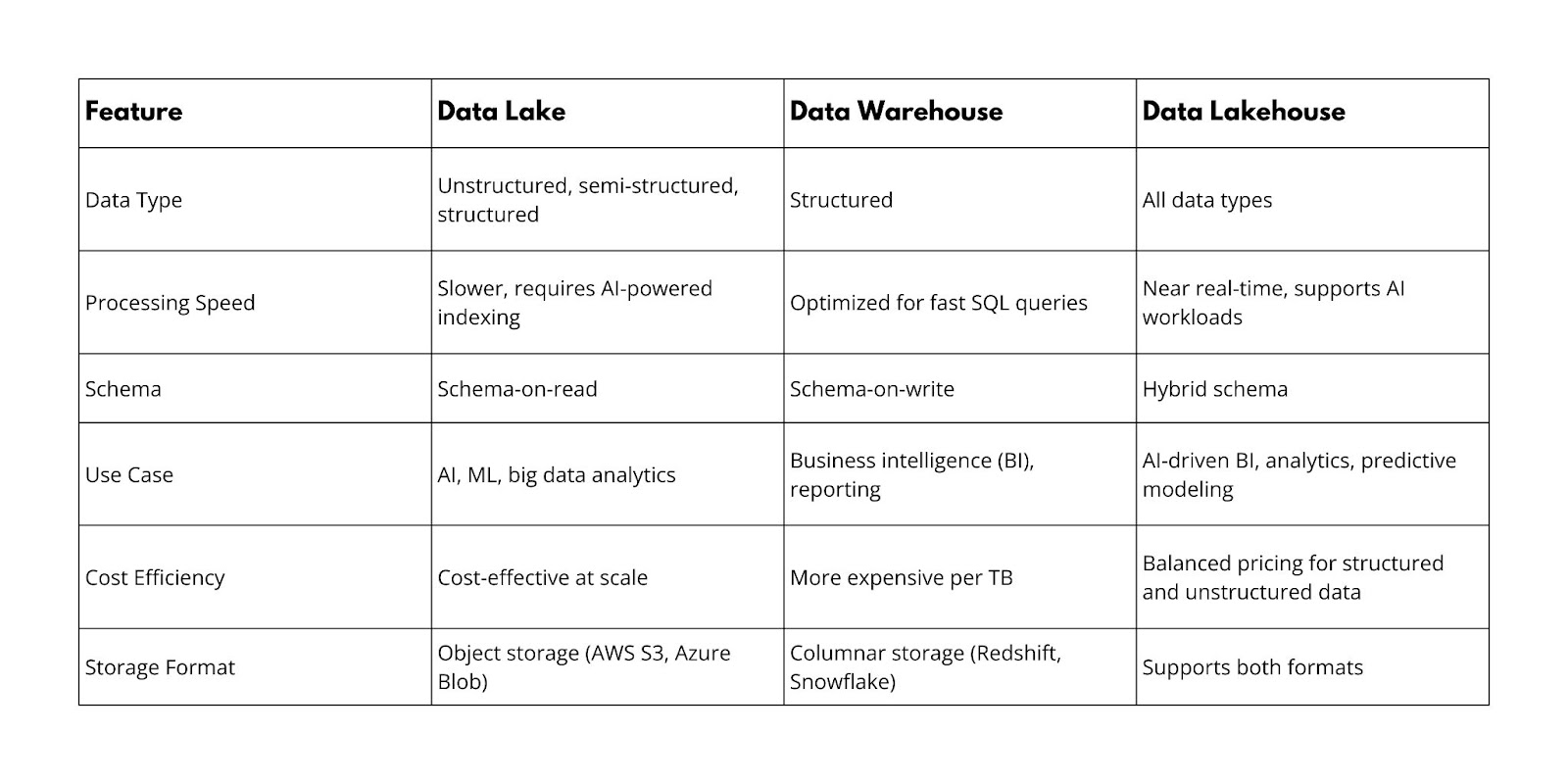
Each of these architectures serves a distinct purpose, and selecting the right one depends on business goals, analytics needs, and compliance requirements.When to use a data lakeA data lake is the best choice for companies handling large volumes of unstructured and semi-structured data, particularly when using AI or machine learning.
- Best for
- AI/ML workloads
- IoT data and sensor analytics
- Natural language processing (NLP) applications
- Predictive modeling
- Example Use Case
- Healthcare organizations use data lakes to store and analyze real-time patient monitoring data, enabling early disease detection and AI-assisted diagnosis.
When to use a data warehouse
A data warehouse is ideal for businesses focused on structured data, business intelligence (BI), and historical reporting.
- Best for
- Financial reporting and compliance
- Supply chain optimization
- Customer relationship management (CRM) analytics
- Retail sales forecasting
- Example Use Case
- Banks and financial institutions rely on data warehouses for secure transaction history analysis, ensuring compliance with regulations like GDPR and SOC 2.
When to use a data lakehouse
The data lakehouse combines the flexibility of data lakes with the structured processing power of warehouses. This hybrid model is increasingly popular among AI-driven enterprises.
- Best for
- AI-powered decision-making
- Advanced data science and big data analytics
- Predictive insights at scale
- Real-time processing with structured and unstructured data
- Example Use Case
- E-commerce giants like Shopify use data lakehouses to optimize pricing strategies by analyzing real-time website traffic, historical sales, and competitor pricing trends simultaneously.
Future-proofing your data strategy with the right choice
According to Forrester Research, by 2026, 75% of businesses will use a hybrid data lakehouse model to support AI-driven analytics. With AI adoption accelerating, organizations must ensure their data architecture aligns with evolving business needs.
- For AI-heavy industries like healthcare, manufacturing, and IoT, a data lake is essential.
- For BI-driven industries like finance and retail, a data warehouse provides stability and structured insights.
- For businesses looking to leverage AI for predictive analytics, a data lakehouse delivers the best of both worlds.
How to get started
The right data infrastructure depends on the business’s data maturity level. Companies looking to adopt AI and automation must consider scalable cloud solutions like:
- AWS Lake Formation for building secure, AI-driven data lakes.
- Amazon Redshift for high-performance data warehousing.
- Databricks Lakehouse for seamless AI analytics across structured and unstructured data.
5. Navigating security, governance, and compliance in data storage
As businesses amass vast amounts of data, security, governance, and compliance have become critical factors in choosing between data lakes and data warehouses. A poorly governed data infrastructure can lead to financial losses, regulatory fines, and data breaches. This section explores how organizations can navigate these challenges while maintaining a secure and AI-ready data environment.
Why security matters more than ever
- The cost of a data breach is rising – In 2023, the average data breach cost reached $4.45 million, a 15% increase over the past three years.
- SMBs are prime targets – 43% of cyberattacks now target small and medium businesses, many of which lack enterprise-grade security.
- AI-driven security threats – Malicious AI is increasingly used to automate cyberattacks, creating sophisticated phishing campaigns and real-time exploits.
Given these risks, organizations must adopt cloud-based security solutions that offer AI-driven threat detection, compliance automation, and real-time monitoring.Compliance: a dealbreaker for data storageDifferent industries face stringent regulatory frameworks regarding data handling. Failing to comply can result in hefty penalties and reputational damage.

Organizations handling sensitive financial, healthcare, or personal data must ensure their chosen data storage architecture meets these compliance requirements.
Data lake vs. data warehouse: which is more secure?

While data warehouses inherently provide stronger governance and security, data lakes can be secured effectively with cloud-based security frameworks.
How to secure your data lake or warehouse
Regardless of whether an organization chooses a data lake, data warehouse, or a hybrid lakehouse, security best practices must be prioritized.
- Implement role-based access control (RBAC)
- Restrict access to sensitive data based on user roles and responsibilities.
- Use AWS Identity and Access Management (IAM) for fine-grained access controls.
- Encrypt data at rest and in transit
- Ensure end-to-end encryption using AWS Key Management Service.
- Adopt TLS encryption for secure data transfers.
- Monitor for anomalies using AI-driven security tools
- AI-based security tools like Amazon GuardDuty analyze traffic patterns and detect potential security threats.
- Ensure automated compliance tracking
- Use AWS Audit Manager to continuously assess regulatory compliance.
- Adopt a zero-trust security framework
- Require authentication at every level, ensuring no implicit trust is granted within the network.
The role of AI in data security and governance
As data volumes grow, organizations must leverage AI-powered security solutions to enhance governance and risk management.
- AI-driven anomaly detection – Machine learning models analyze behavioral patterns, identifying suspicious activities in real time.
- Automated compliance reporting – AI tools generate real-time compliance insights, reducing manual efforts.
- Predictive security analytics – AI models forecast potential cyberattack patterns, allowing businesses to proactively strengthen defenses.
6. Cloud-based data solutions—why migration is inevitable
As enterprises continue their digital transformation journeys, migrating to cloud-based data solutions has become an imperative rather than an option. Businesses relying on on-premise data storage solutions face challenges such as scalability limitations, high maintenance costs, and outdated security frameworks. The shift toward cloud-native data lakes and data warehouses is reshaping how organizations store, process, and utilize data for real-time insights.
The global push toward cloud-first data strategies
- By 2026, 75% of all enterprise data will be created and processed in the cloud rather than traditional data centers.
- 96% of businesses have already adopted cloud computing in some form, with 50% using multi-cloud strategies to optimize workloads.
- Organizations migrating from on-prem to cloud-based data lakes experience up to a 60% reduction in data management costs.
With AI, analytics, and automation driving next-gen business strategies, cloud-based data architectures provide the agility needed to remain competitive.
Why on-premise data storage is becoming obsolete
While on-premise data warehouses were once the gold standard for structured data storage, they are failing to meet the demands of modern businesses due to:
- High capital expenditure (CapEx) – Requires significant upfront investments in hardware, networking, and maintenance.
- Limited scalability – Expanding storage capacity requires physical upgrades, making scaling time-consuming and costly.
- Security risks – Traditional data centers are more vulnerable to ransomware attacks and physical security breaches.
- Lack of AI-readiness – On-premise architectures struggle to process AI-driven workloads efficiently, limiting real-time analytics capabilities.
Cloud-based data solutions: advantages over legacy storage

Migrating to AWS for future-proof data storage
Amazon Web Services (AWS) offers enterprise-grade cloud data solutions that address the limitations of legacy storage. Organizations migrating to AWS experience:
- 50% lower operational costs compared to on-premise data centers (AWS Economics Center).
- 3x faster time-to-insights, with services like AWS Redshift and AWS Athena (451 Research).
- Enterprise-grade security with AWS IAM, encryption tools, and AI-driven anomaly detection.
Key AWS services for cloud-based data architecture:
- Amazon S3 – Scalable storage for structured and unstructured data, ideal for data lakes.
- Amazon Redshift – Cloud-based data warehouse optimized for high-speed analytics.
- AWS Lake Formation – Enables businesses to set up secure data lakes in a few clicks.
- AWS Glue – A serverless data integration service that automates data preparation for analytics and machine learning.
For businesses looking to modernize their data infrastructure while enhancing AI-readiness, our Cloud Services help organizations navigate seamless cloud migration, data security, and AI-powered insights.
Key considerations when migrating to cloud-based data lakes and warehouses
To ensure a successful migration, businesses should:
- Assess data storage needs – Identify structured, semi-structured, and unstructured data types.
- Define security & compliance requirements – Ensure regulatory compliance for GDPR, HIPAA, or PDPA.
- Optimize cloud costs – Use AWS Cost Explorer to forecast cloud expenses.
- Leverage AI-powered automation – Integrate AWS Glue and QuickSight for real-time analytics.
- Choose the right migration strategy – Opt for rehosting, refactoring, or re-platforming based on business needs.
7. AI-powered data lakes & warehouses—unlocking the future of intelligent analytics
With exponential data growth and the increasing complexity of business operations, AI-powered data lakes and warehouses are revolutionizing how companies store, process, and analyze information. Traditional data architectures, reliant on manual indexing and rule-based processing, struggle to keep up with modern AI-driven applications. Organizations that integrate AI-powered analytics into their cloud data strategies gain significant competitive advantages, including predictive insights, automated decision-making, and enhanced efficiency.
The shift toward AI-driven data architectures
- By 2025, AI-powered data management will automate 75% of enterprise data processing tasks, reducing manual intervention and improving decision accuracy.
- AI-enabled data warehouses reduce query processing time by up to 90%, improving operational efficiency in industries such as finance, healthcare, and retail.
- AI-powered analytics increases decision-making speed by 5x, enabling real-time insights for dynamic business environments.
The integration of AI and ML models with cloud-based data lakes allows businesses to predict trends, detect anomalies, and automate data classification, unlocking new revenue opportunities and enhancing customer experiences.
How AI enhances data lakes & warehouses
AI-driven data architectures automate and optimize processes across three key areas:
1. Automated data ingestion & classification
- Traditional data ingestion requires manual formatting and categorization. AI automates data ingestion pipelines, classifying structured and unstructured data in real-time.
- Example: AWS Glue uses AI-based schema detection to categorize incoming data streams, reducing processing time by 40%.
2. Predictive analytics & real-time insights
- AI-driven predictive analytics models process massive datasets to forecast market trends, sales demand, and customer behavior.
- Example: AI-enhanced warehouses like Amazon Redshift ML allow businesses to integrate machine learning models directly into SQL queries for real-time insights.
3. Intelligent data security & anomaly detection
- AI strengthens data security by identifying irregular access patterns and potential cyber threats in real-time.
- Example: AWS Macie leverages AI-powered anomaly detection to classify sensitive data and flag unauthorized access attempts, reducing security risks by 50%.
Real-world use cases of AI-driven data lakes & warehousesFinance: AI-powered fraud detection & risk analysis
- Banks using AI-enabled cloud warehouses detect fraud up to 60% faster by analyzing transactional patterns.
- AWS AI-powered fraud detection systems flag unusual transactions, reducing financial losses.
Healthcare: predictive diagnostics & patient data management
- AI-based data lakes process electronic health records (EHRs), enabling early disease detection and personalized treatment recommendations.
- AWS HealthLake integrates AI to extract meaningful insights from unstructured medical data, accelerating diagnosis and drug research.
Retail: customer behavior insights & demand forecasting
- AI-powered warehouses improve inventory forecasting by 30%, reducing overstocking and lost sales.
- AWS Amazon Forecast uses machine learning to predict demand fluctuations based on consumer behavior and market trends.
Leveraging AI-driven analytics with AWS data solutions
AWS provides cutting-edge AI-driven tools for enhancing data lakes and warehouses:
- Amazon Redshift ML – Embeds machine learning models into data warehouses for predictive insights.
- AWS Glue DataBrew – Automates data cleansing and transformation, reducing manual processing effort.
- Amazon Lookout for Metrics – Detects business anomalies in real-time using AI-driven monitoring.
- AWS Macie – Enhances data security with AI-powered risk analysis.
8. Choosing the right data strategy—when to use a data lake vs. a data warehouse
As businesses generate and collect unprecedented volumes of data, choosing between a data lake and a data warehouse is a strategic decision that impacts performance, costs, and analytics capabilities. While both serve as foundational components of modern data architecture, their ideal use cases vary based on storage needs, data types, and analytical goals.By 2026, 90% of large enterprises will have adopted both data lakes and data warehouses in hybrid architectures to balance cost, flexibility, and speed.
When to choose a data lake
A data lake is ideal when:
- The organization deals with large-scale raw data that needs flexibility for AI and ML applications.
- Real-time analytics and predictive modeling are crucial to business operations.
- The business needs cost-effective storage for unstructured and semi-structured data (e.g., social media logs, IoT device data, video/audio files).
Real-world use case: AI-driven financial risk analysis
- Financial institutions use data lakes for fraud detection, analyzing real-time transactional data to identify suspicious activities before they escalate.
- AWS Amazon S3 and AWS Glue allow seamless integration of unstructured financial data for machine learning-driven risk analysis (AWS).
When to choose a data warehouse
A data warehouse is best suited when:
- Data is structured and used for operational analytics or regulatory reporting.
- Fast query performance is required for business intelligence (BI) and KPI tracking.
- The organization relies on historical trend analysis to make strategic decisions.
Real-world use case: optimizing e-commerce analytics
- Online retailers store structured customer transaction data in a data warehouse to analyze buying patterns and improve personalized marketing.
- AWS Amazon Redshift helps e-commerce brands process millions of transactions in seconds, optimizing customer segmentation and marketing automation.
Hybrid approach: when to integrate both data lakes & data warehouses
Many organizations adopt a hybrid strategy, leveraging the scalability of data lakes for big data while using data warehouses for high-performance business intelligence analytics.
- Example: A healthcare provider uses a data lake to store unstructured patient records and medical imaging but integrates it with a data warehouse to generate structured reports for patient treatment outcomes and compliance audits.
- AWS Lake Formation enables companies to integrate data lakes with Redshift for a unified analytics architecture, balancing cost, security, and accessibility (AWS).
9. Future-proofing your data infrastructure—what's next for data lakes & warehouses?
As businesses continue to evolve in the AI-driven digital era, data lakes and data warehouses are becoming more advanced, integrating cloud, automation, and real-time analytics. Organizations must future-proof their data strategies to remain competitive and scalable.Emerging trends shaping the future of data lakes and data warehouses
1. AI and automation-driven data management
- AI-driven data governance tools will automate data classification, compliance monitoring, and anomaly detection in both data lakes and warehouses.
- Generative AI is expected to enhance query optimization and data structuring, reducing manual processing efforts.
- By 2027, over 75% of enterprises will deploy AI-driven data management systems to enhance data integrity and accessibility (Gartner).
- AWS Glue and AI-powered Amazon Redshift ML enable automated ETL (Extract, Transform, Load) processes, making data querying more intelligent and responsive (AWS).
2. Real-time data streaming for decision-making
- Businesses are increasingly shifting towards real-time data analytics to improve decision-making, customer experiences, and fraud detection.
- Apache Kafka, AWS Kinesis, and Snowflake Streaming are accelerating real-time data ingestion and analysis, reducing dependency on static, batch-processed data.
- By 2026, over 65% of enterprises will integrate real-time data analytics into their business operations (Forrester).
- Example: Financial services firms leverage AWS Kinesis for instant fraud detection, analyzing billions of transactions within seconds.
3. Convergence of data lakes and warehouses—“lakehouse” architecture
- The lakehouse model combines the best of data lakes and data warehouses, enabling structured querying over large, flexible datasets.
- Databricks and AWS Lake Formation are leading innovations in unified data architectures that offer the scalability of data lakes with the structured querying efficiency of warehouses.
- By 2028, lakehouse architectures will power over 50% of enterprise data infrastructures (IDC).
- Example: Companies using AWS Lake Formation + Redshift can now store semi-structured IoT data in S3 while querying it seamlessly through SQL-based analytics.
4. Cloud-native, serverless, and multi-cloud strategies
- More enterprises are adopting serverless and multi-cloud architectures to enhance scalability, performance, and cost efficiency.
- AWS Redshift Serverless, Google BigQuery, and Snowflake Cloud allow businesses to run analytics workloads without infrastructure management.
- By 2025, 60% of data workloads will be cloud-native, eliminating traditional on-premise constraints (McKinsey).
- Example: With AWS Redshift Serverless, companies run complex analytical queries instantly while only paying for the compute resources used.
5. Sustainability and green data architecture
- With rising environmental concerns, cloud providers are optimizing data storage solutions to reduce carbon footprints.
- AWS and Google Cloud are developing carbon-aware cloud regions, ensuring businesses can track and minimize their data processing emissions.
- By 2030, sustainable cloud data centers will reduce global energy consumption for data management by 40% (World Economic Forum).
- Example: AWS’s Graviton-powered instances consume 60% less energy than traditional server-based data processing.
Navigating the futureAs data lakes and warehouses evolve, businesses must adapt to AI-driven, real-time, and scalable architectures. Whether your organization is looking to optimize data analytics, integrate AI-driven automation, or transition to a serverless environment, our AI & Data Services can help build future-ready, cloud-native solutions.